In a rapidly evolving digital landscape, autonomous vehicle technologies have emerged as a cornerstone of innovation. Advancements in perception and localization systems are revolutionizing navigation, making self-driving cars safer and more efficient. Vraj Mukeshbhai Patel, an expert in autonomous mobility, explores the integration of cutting-edge technologies in this field. His insights highlight how sensor fusion, high-definition mapping, and machine learning propel autonomous systems toward higher levels of accuracy and reliability.
From GPS-IMU to Multi-Modal Integration
Early autonomous navigation relied heavily on GPS-IMU systems, which faced limitations in urban environments with signal obstructions and drift errors. The transition to tightly coupled sensor fusion has significantly improved positioning accuracy. Modern systems achieve submeter accuracy even in challenging terrains by incorporating multiple global navigation satellite systems (GNSS) and adaptive filtering algorithms. The integration of vision-aided inertial navigation has further refined localization, reducing errors and enhancing performance in real-world conditions. Additionally, high-frequency updates and real-time error corrections provide greater consistency in vehicle tracking.
The Power of High-Definition Mapping
High-definition (HD) maps serve as the backbone of precise autonomous navigation. These maps leverage LiDAR-based point cloud data and real-time kinematic GPS to achieve centimeter-level accuracy. Advanced semantic labeling techniques accurately classify road elements, improving object detection and scene understanding. The multi-layered mapping approach categorizes static and dynamic elements, helping vehicles adapt to varying road conditions. Additionally, dynamic map updates ensure that autonomous cars can adjust to real-time infrastructure changes, making navigation more robust and dependable. HD mapping enhances localization accuracy and provides critical data for predictive analytics in route planning.
Multi-Modal Sensor Fusion: A Game Changer
Modern autonomous vehicles integrate diverse sensor modalities, including LiDAR, radar, and high-resolution cameras, to enhance situational awareness. Sensor fusion frameworks synchronize data streams, optimizing spatial and temporal alignment for improved detection and classification. These systems exhibit remarkable resilience against environmental variables, maintaining high accuracy even in adverse weather conditions such as heavy rain and fog. The ability to seamlessly merge multiple sensor inputs strengthens the reliability of perception systems, reducing false positives and ensuring safer navigation. Moreover, advancements in deep learning models enable the system to distinguish between static and moving objects, significantly improving object tracking capabilities.
Machine Learning in Environmental Perception
Deep learning algorithms play a crucial role in enhancing autonomous perception. Neural networks trained on vast datasets enable vehicles to recognize objects, predict trajectories, and respond to dynamic scenarios. Transformer-based motion prediction models enhance behavioral analysis, allowing autonomous systems to anticipate movements and make informed decisions. These innovations significantly improve obstacle avoidance and real-time adaptation in complex urban settings. Additionally, continuous learning mechanisms enable autonomous systems to refine their predictive models, adapting to new driving conditions and evolving traffic patterns.
Edge Computing for Real-Time Decision Making
The implementation of edge computing in autonomous vehicles has redefined real-time processing capabilities. Edge architectures reduce latency by decentralizing computation and optimizing data flow, enabling instantaneous decision-making. Intelligent workload distribution ensures optimal resource utilization, enhancing efficiency while maintaining energy constraints. The integration of predictive analytics further strengthens system responsiveness, paving the way for more autonomous and self-reliant vehicles. Additionally, real-time monitoring and self-diagnosis capabilities allow immediate system recalibration, ensuring continued operational reliability and safety.
Paving the Way to Full Autonomy
As autonomous technology advances, achieving Level 4 and 5 autonomy becomes more feasible. Enhanced safety architectures, rigorous validation, and real-world testing have improved reliability across diverse environments. Machine learning and next-gen AI continue to drive progress, while evolving regulations ensure safe deployment. Overcoming remaining challenges will require further sensor integration and ethical considerations, paving the way for fully autonomous systems in real-world conditions.
In conclusion, Vraj Mukeshbhai Patel highlights the transformative impact of perception and localization advancements in autonomous vehicles. The industry is making significant strides toward safer and more intelligent autonomous mobility by leveraging multi-modal sensor fusion, high-definition mapping, and machine learning. As innovations continue to refine these technologies, the vision of fully autonomous transportation is steadily becoming a reality, promising a future where self-driving systems seamlessly integrate into everyday life. The continued development of these innovations will play a crucial role in reshaping urban mobility, improving traffic efficiency, and reducing environmental impact through optimized navigation solutions.
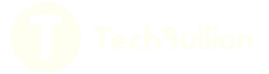