The healthcare industry is undergoing a significant transformation, powered by innovations in real-time clinical decision systems and machine learning-driven optimization. These advancements are helping providers improve patient outcomes and streamline healthcare delivery. Mohit Agarwal’s recent exploration of these systems delves into the technical infrastructure, applications, and challenges healthcare organizations face when implementing these cutting-edge technologies.
Harnessing the Power of Machine Learning
Machine learning (ML) has emerged as a cornerstone of healthcare transformation. In clinical settings, ML models are increasingly deployed to enhance diagnostic accuracy, optimize treatment plans, and even predict health risks. These systems use vast amounts of data—ranging from medical images to patient history and real-time vital signs—to identify patterns that signal emerging health issues. ML-powered decision support tools are now capable of predicting diseases such as sepsis and heart attacks, enabling faster interventions and better outcomes.
These systems also revolutionize the management of chronic conditions by providing real-time insights into patients’ health status. Moreover, ML algorithms improve personalized treatment recommendations by analyzing genetic data, medical history, and lifestyle factors.
Advancing Technical Infrastructure
A robust technical infrastructure is essential to support the real-time data processing required in modern healthcare systems. Healthcare organizations now rely on a combination of electronic health records (EHRs), wearable devices, and cloud-based platforms to manage the massive volumes of data generated daily. The challenge lies in ensuring that these systems can seamlessly integrate, process, and analyze data from various sources without compromising security or speed.
Distributed computing systems, along with edge computing nodes, meet the demands of real-time data processing. These systems allow for immediate analysis of data from devices like heart monitors, which is especially critical in intensive care units where seconds matter. Hospitals can now ensure that healthcare providers have access to accurate patient data.
Revolutionizing Clinical Applications
Incorporating advanced clinical applications into healthcare systems has had a profound impact on the way care is delivered. Predictive diagnostics, personalized medicine, and resource optimization are key areas where innovations are making a difference.
For example, predictive diagnostic systems have evolved into tools that analyze multiple parameters to identify potential issues before they become critical. Hospitals can now detect early warning signs of conditions such as cancer or cardiac events, leading to earlier interventions and better survival rates.
Furthermore, precision medicine is rapidly gaining traction in oncology, where ML algorithms recommend the most effective treatment plans based on genetic profiles. This shift improves outcomes and reduces the trial-and-error process.
Overcoming Implementation Challenges
Despite the tremendous potential of these technologies, healthcare providers face challenges when implementing real-time clinical decision systems. One of the foremost obstacles is ensuring the privacy and security of patient data. Healthcare systems are frequent targets of cyber-attacks, and organizations must invest in robust cybersecurity measures to protect against breaches.
Another significant challenge is the need for model interpretability. ML algorithms, particularly in clinical decision support systems, must provide transparent reasoning behind their recommendations. Healthcare providers must trust these systems, and ensuring their explanations is crucial for adoption by clinicians.
Looking Ahead: Future Opportunities in Healthcare
The future of healthcare is poised to be even more transformative as emerging technologies such as quantum computing and next-generation biosensors come into play. These advancements promise to improve disease detection rates, reduce diagnostic errors, and enhance resource allocation.
As these technologies evolve, healthcare organizations must focus on refining their integration strategies, ensuring innovations are implemented in ways that are both practical and compliant with regulatory standards. The use of big data analytics will enable healthcare systems to shift from a reactive model to a proactive approach, focusing more on prevention and early intervention.
In conclusion, the integration of real-time clinical decision systems and machine learning-driven optimization has brought about a paradigm shift in healthcare delivery. These innovations are enhancing patient care, improving operational efficiency, and ensuring more accurate diagnoses and treatment plans. However, as Mohit Agarwal emphasizes, successful adoption of these technologies requires overcoming challenges related to data security, model interpretability, and algorithmic bias. With careful planning, investment in infrastructure, and a focus on patient safety, the healthcare sector is poised for a new era of innovation and better outcomes for all.
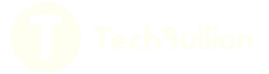