In this digital world, artificial intelligence (AI) is revolutionizing industries, bringing both opportunities and challenges. The growing adoption of generative AI has necessitated robust governance frameworks to ensure data security, model transparency, and ethical implementation. Abhilash Nagilla, a researcher in AI governance, explores the evolution of AI governance frameworks and their impact on modern AI applications. His insights emphasize the necessity of structured governance approaches to enhance AI deployments and regulatory compliance.
The Evolution of AI Governance
Traditional data governance models are no longer sufficient for managing AI-driven applications. With the complexity of machine learning models, organizations face challenges in ensuring data integrity, privacy, and security. The shift from traditional governance to AI-specific frameworks is evident, with organizations implementing dedicated AI governance policies to manage model risks and improve reliability. AI governance now focuses on fairness, accountability, and human oversight, aligning with emerging regulations and ethical standards.
Strengthening Data Quality Management
Effective AI governance begins with robust data quality management. Organizations implementing structured data validation protocols have reported significant improvements in model accuracy and reliability. Advanced techniques such as real-time data lineage tracking and automated data drift monitoring enhance AI system performance and compliance. Additionally, synthetic data governance frameworks have emerged as a critical component, reducing data privacy risks while maintaining model effectiveness.
Reinforcing Security and Privacy Controls
AI security has become a primary concern for organizations adopting generative AI models. Implementing multi-layered security protocols significantly reduces data breaches and unauthorized model interactions. Encryption standards, model access governance, and stringent security policies are essential in protecting AI architectures. Moreover, organizations that integrate AI-specific regulatory compliance measures experience substantial improvements in security posture and audit outcomes.
Advancing Ethical AI Practices
AI bias and fairness are crucial considerations in governance frameworks. Automated bias detection mechanisms enhance ethical AI decision-making, reducing algorithmic discrimination. Organizations implementing ethical AI principles see higher stakeholder trust and compliance with fairness guidelines. Ensuring AI output authenticity through watermarking and verification systems further strengthens transparency and credibility in AI-generated content.
Continuous Monitoring and Adaptation
The ever-evolving nature of AI systems necessitates continuous monitoring and adaptive governance to ensure reliability, security, and compliance. Real-time performance tracking allows organizations to detect anomalies early and implement necessary corrections, preventing potential failures. By integrating structured feedback mechanisms, AI models can be fine-tuned for greater accuracy and fairness, enhancing stakeholder trust. Regularly updating governance policies ensures that organizations remain compliant with evolving regulations and emerging risks, fostering a proactive approach to ethical AI deployment and responsible innovation.
The Impact of Governance on AI Success
Effective AI governance frameworks significantly enhance AI investments and operational efficiency, delivering higher returns while reducing compliance costs and mitigating risks. Organizations with well-structured governance strategies benefit from improved regulatory adherence, stronger security measures, and ethical AI implementation. AI governance ensures transparency, accountability, and trust across industries, safeguarding against biases and security threats. Scalable governance models allow organizations to adapt AI policies across different applications, ensuring flexibility in an evolving technological landscape. As AI adoption grows, a structured governance approach becomes essential for sustainable innovation, regulatory compliance, and the responsible deployment of AI solutions in various sectors.
Future of AI Governance
The future of AI governance depends on adaptive and scalable frameworks that can seamlessly evolve alongside technological advancements. With AI systems becoming increasingly autonomous, organizations must establish proactive governance strategies to address emerging risks. One key innovation is the integration of blockchain for immutable audit trails, ensuring transparency and accountability in AI-driven decisions. Additionally, explainable AI (XAI) mechanisms will enhance interpretability, enabling organizations to understand and justify AI outcomes. Automated compliance verification will streamline regulatory adherence, reducing operational risks and ensuring ethical AI implementation. As AI governance frameworks become more dynamic, businesses must prioritize continuous monitoring, bias mitigation, and robust security measures to foster stakeholder trust while maintaining compliance with evolving global AI regulations.
In conclusion, Abhilash Nagilla highlights the necessity of robust AI governance frameworks to navigate the complexities of modern AI applications. As AI continues to reshape industries, structured governance will be the key to achieving innovation with responsibility, ensuring trust and compliance in AI-driven transformations.
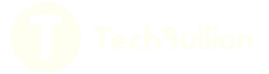