In this modern era, where edge computing is becoming increasingly crucial, Dileep Kumar Reddy Lankala, an expert in computer science, presents a groundbreaking approach to observability in edge computing environments. His latest research introduces a lightweight framework that enhances system monitoring without overburdening resources.
The Challenge of Observability in Edge Computing
With the rapid growth of the Internet of Things (IoT), traditional cloud-based monitoring methods are struggling to keep up. Edge computing, which processes data closer to its source, offers a solution but ensuring system observability while maintaining performance remains a challenge. Conventional monitoring tools often consume excessive resources, leading to increased latency and energy consumption.
A Smarter Monitoring Framework
The proposed framework is designed to provide efficient system visibility without overloading edge devices. It introduces adaptive sampling techniques that dynamically adjust data collection based on system conditions. This significantly reduces data transmission rates while ensuring critical events are detected in real time.
Processing Data at the Edge
One key innovation of this framework is edge-local processing, allowing data to be analyzed closer to where it is generated. This minimizes the need for continuous cloud communication, reducing network congestion and improving response times. Optimized machine learning models run directly on edge devices, ensuring accurate anomaly detection with minimal resource consumption.
Optimized Log Aggregation
Traditional logging methods can generate overwhelming amounts of data, which is impractical for edge environments. The new framework employs intelligent compression algorithms and prioritization techniques, allowing logs to be stored efficiently without compromising insight. This ensures only relevant data is transmitted while maintaining robust historical records.
Enhanced Resource Management
Edge devices operate with limited processing power, memory, and battery life. The framework’s resource-aware design ensures monitoring operations remain lightweight, consuming as little as 2% of available resources. Dynamic workload balancing optimizes performance, adjusting monitoring levels based on real-time system health.
Real-World Impact
This innovation has demonstrated remarkable results in various applications. In smart agriculture, it has improved data collection reliability while reducing network bandwidth usage. In industrial automation, the framework has significantly enhanced fault detection accuracy while minimizing false alarms. These improvements translate to better efficiency, reduced costs, and more sustainable IoT deployments.
Security and Privacy Considerations
As edge computing evolves, security and data privacy remain crucial concerns. The framework incorporates end-to-end encryption and secure authentication protocols to protect sensitive data. Additionally, it employs federated learning techniques to train machine learning models without transmitting raw data to the cloud, further enhancing privacy and security.
Future Enhancements and Expansions
Looking ahead, the framework aims to integrate advanced artificial intelligence techniques for predictive analytics and self-healing capabilities. This will allow systems to anticipate failures before they occur and take proactive measures to mitigate risks. Ongoing research focuses on enhancing interoperability with existing IoT infrastructures, ensuring seamless integration across different platforms and industries.
Scalability and Adaptability
A standout feature of the framework is its scalability. Whether deployed in a smart home system or a large industrial IoT network, it adapts to varying workloads and resource constraints. Its modular architecture allows developers to customize functionalities based on specific needs, making it a versatile solution for diverse applications. This adaptability ensures enterprises of all sizes can benefit from advanced observability, increasing operational efficiency and cost savings.
Expanding Industry Applications
Beyond agriculture and industrial automation, the framework has applications in smart healthcare, transportation, and energy management. In healthcare, it enhances patient monitoring systems by ensuring real-time anomaly detection. In transportation, it optimizes traffic flow by analyzing vehicular data efficiently. The energy sector benefits from improved monitoring of distributed grids, leading to better energy distribution and sustainability. These possibilities highlight the framework’s potential in transforming various industries.
In conclusion, Dileep Kumar Reddy Lankala’s research sets a new standard for monitoring in edge computing environments. By leveraging adaptive sampling, edge-local processing, and efficient log aggregation, this framework provides a scalable, efficient, and reliable solution for IoT observability. As the demand for smarter and more resource-efficient computing grows, this innovation will play a crucial role in shaping the future of connected systems. With further research and integration, it has the potential to revolutionize multiple industries and create a smarter, more connected world.
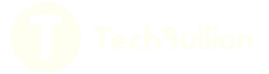