In an era where website reliability dictates business success, innovative maintenance solutions are reshaping the digital landscape. Shailesh Kumar Agrahari, an expert in AI-driven development, explores how predictive maintenance is transforming website performance, security, and operational efficiency. His insights highlight the crucial role of artificial intelligence in preventing failures before they occur, ensuring seamless digital experiences for users.
The Rise of Predictive Maintenance
Traditional website maintenance relied on reactive strategies, often leading to unplanned downtime and escalating costs. Predictive maintenance, powered by AI, introduces a proactive approach by leveraging machine learning algorithms to detect anomalies before they disrupt operations. Organizations that adopt AI-driven maintenance have reported up to a 50% reduction in website downtime and a 30% decrease in maintenance costs.
Advanced Data Collection and Monitoring
The backbone of predictive maintenance lies in its ability to collect and analyze vast amounts of operational data. AI-powered monitoring tools can process up to 1.5 terabytes of data daily, identifying inefficiencies in real time. This results in optimized resource utilization, with organizations witnessing a 25–35% improvement in server efficiency. Additionally, memory optimization through predictive analytics has been shown to reduce application crashes by 40%, ensuring smoother website performance.
Machine Learning for Intelligent Predictions
The integration of machine learning pipelines enhances predictive maintenance by refining data quality and improving early failure detection. Advanced AI models, such as deep learning networks, achieve an impressive 92–95% accuracy in predicting website failures. Long short-term memory (LSTM) models, for instance, can anticipate technical issues up to 72 hours in advance, significantly reducing downtime risks.
Anomaly Detection: Strengthening Website Security
Beyond maintenance, predictive analytics plays a pivotal role in website security. AI-powered anomaly detection systems process thousands of data points per second, identifying irregular patterns with up to 93% accuracy. These systems integrate statistical analysis and machine learning to reduce false positives by 65%, enabling businesses to detect and mitigate cyber threats before they escalate. This proactive approach to cybersecurity not only minimizes potential damage but also significantly reduces response time, allowing security teams to focus on genuine threats rather than investigating false alarms.
Automating Root Cause Analysis
One of the most significant advancements in AI-driven maintenance is its ability to automate root cause analysis. Traditional troubleshooting methods often require manual intervention, increasing response time and operational costs. AI-based root cause analysis can process over one million events per second, reducing mean time to resolution (MTTR) by 80%. This automation allows businesses to swiftly identify and resolve issues, improving overall website reliability.
Enhancing Performance with AI-Driven Optimization
AI doesn’t just predict failures—it actively enhances website performance. AI-powered optimization tools have led to a 40% reduction in server response times and a 35% improvement in page load speeds. By analyzing user behavior and technical metrics, AI systems automatically adjust resource allocation, ensuring a seamless browsing experience.
Resource Optimization for Cost Efficiency
Organizations implementing AI-driven predictive maintenance report significant cost savings through efficient resource management. Studies show that machine learning-based inventory optimization can reduce maintenance-related costs by up to 25%. Additionally, predictive analytics enhances spare parts forecasting with 94% accuracy, minimizing unnecessary expenses.
Future Developments in AI Maintenance
The evolution of predictive maintenance continues, with AI innovations driving even greater efficiency. Future AI models aim to achieve over 95% accuracy in failure prediction while reducing overall maintenance costs by 30–40%. As automation capabilities expand, businesses can expect faster incident response times and improved fault diagnosis, revolutionizing how digital infrastructures are maintained. These advancements in AI technology will enable more sophisticated pattern recognition and anomaly detection, allowing organizations to implement truly autonomous maintenance systems that can self-diagnose and initiate corrective actions with minimal human intervention.
In conclusion ,the integration of AI into predictive maintenance is transforming website reliability, security, and performance. By leveraging machine learning, anomaly detection, and automated root cause analysis, businesses can transition from reactive to proactive maintenance strategies. Shailesh Kumar Agrahari’s insights underline the immense potential of AI-driven maintenance in ensuring seamless digital experiences while significantly reducing operational costs. As technology continues to advance, AI-powered predictive maintenance will set new industry standards, paving the way for a smarter, more efficient digital future.
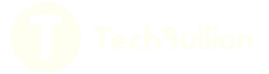