In the evolving landscape of data-driven decision-making, Enterprise Data Engineering has emerged as a cornerstone of modern business strategies. Dheeraj Kumar Bansal, an expert in data architecture, explores innovations shaping the future of data warehousing, highlighting advancements in data integration, architecture optimization, automation, scalability, and real-time processing that redefine how organizations leverage information effectively.
Transforming Data Integration for Business Insights
Integrating diverse data sources is fundamental to Enterprise Data Engineering. Organizations collect vast amounts of information from point-of-sale systems, customer relationship management platforms, e-commerce portals, and loyalty programs. The challenge lies in harmonizing these disparate sources into a unified system that offers valuable insights.
Modern Extract, Transform, Load (ETL) and Extract, Load, Transform (ELT) processes play a crucial role in achieving this integration. While traditional ETL methods transform data before loading it into warehouses, ELT leverages cloud-based processing power to transform data post-loading. This shift enables enterprises to handle larger datasets more efficiently.
The Evolution of Data Warehouse Architecture
Data warehouses serve as the backbone of enterprise analytics. The shift from on-premises storage to cloud-based solutions has enabled businesses to scale operations seamlessly. Cloud platforms offer flexible storage options, automated maintenance, and global accessibility, making them a preferred choice for enterprises looking to streamline data management.
However, organizations must weigh trade-offs between cloud and on-premises solutions. While cloud warehouses offer scalability and reduced infrastructure maintenance, on-premises solutions provide greater control over security and customization. Many businesses adopt a hybrid model that combines the strengths of both approaches.
Optimizing Performance Through Dimensional Modeling
Dimensional modeling remains a critical technique for structuring data warehouses effectively. The star and snowflake schemas provide structured frameworks for storing and retrieving data efficiently. Star schemas facilitate faster query performance, whereas snowflake schemas offer improved data normalization and storage optimization.
Fact tables, containing business metrics, and dimension tables, offering contextual data, work together to create an efficient analytical structure. This approach ensures that organizations generate meaningful insights without compromising performance.
Enhancing Analytics with Specialized Data Marts
Data marts have revolutionized how organizations access and analyze data. These specialized repositories serve individual departments, enabling targeted analytics without burdening the central data warehouse. Marketing data marts focus on campaign performance, while finance data marts streamline financial reporting.
By creating department-specific data marts, organizations can enhance query speed and customize data views. This structure prevents data silos while ensuring accessibility across various teams.
The Power of Enterprise Data Warehousing
Enterprise Data Warehousing (EDW) delivers transformative benefits by consolidating data into a single repository. The advantages include:
- A unified view of organizational data, reducing inconsistencies and enabling accurate reporting.
- Scalable architectures accommodating growing data volumes.
- Optimized query execution through indexing, columnar storage, and materialized views.
- Enhanced business intelligence capabilities, driving data-informed decision-making.
As enterprises continue to harness big data, the role of EDW in facilitating seamless analytics and predictive modeling becomes increasingly critical.
Strengthening Data Governance and Security
With rising emphasis on data privacy and compliance, robust data governance practices have become a necessity. Organizations are implementing:
- Automated data quality checks to maintain accuracy.
- Role-based access controls to protect sensitive information.
- Compliance frameworks aligning with regulations.
- Metadata management solutions to track data lineage.
A well-structured governance framework ensures data reliability, reducing risks associated with erroneous or unauthorized access.
Advanced Analytics: AI and Real-Time Processing
The future of data warehousing lies in advanced analytics powered by artificial intelligence (AI) and real-time processing. Enterprises integrate AI-driven automation to streamline data cleansing and anomaly detection, reducing manual effort.
Additionally, real-time analytics enables organizations to derive instant insights, enhancing operational agility. The integration of data lakes alongside traditional warehouses allows businesses to manage both structured and unstructured data.
In conclusion, as the digital landscape evolves, Enterprise Data Engineering stands at the forefront of innovation. The transition towards cloud-based architectures, real-time processing, and AI-driven analytics is redefining how businesses manage data. Dheeraj Kumar Bansal‘s insights underscore the significance of robust data strategies in unlocking enterprise information systems. By adopting cutting-edge data warehousing practices, organizations can drive smarter decision-making, achieve operational excellence, and maintain a competitive edge in the data-driven world.
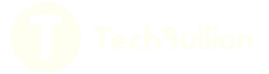