In today’s digital landscape, organizations face the dual challenge of driving technological innovation while safeguarding user privacy. Amber Chowdhary, a notable figure in the field of AI and data privacy, explores this pressing issue in his latest work. His insights delve into the implementation of privacy-first architectures, highlighting advancements in secure data processing, ethical AI development, and regulatory compliance.
With the increase in digital transactions and data-driven decision-making, ensuring privacy has become a primary concern for both organizations and consumers. Companies must adopt comprehensive privacy-first strategies to maintain trust and regulatory compliance. By embedding security measures at every stage of data processing, organizations can prevent breaches, reduce vulnerabilities, and build resilient infrastructures that protect user data from unauthorized access.
Revolutionizing Data Protection Mechanisms
The evolution of data protection infrastructure has led to groundbreaking methodologies for securing personal information. Traditional data security focused primarily on encryption, but modern solutions now integrate advanced anonymization and pseudonymization techniques. Differential privacy algorithms and homomorphic encryption are revolutionizing the field, allowing organizations to use data without exposing sensitive information. Hybrid encryption schemes, which combine symmetric and asymmetric encryption, ensure optimal security without compromising system efficiency. The incorporation of zero-trust architectures further strengthens protection by verifying every data transaction before granting access.
Ethical AI: A Framework for Fairness and Transparency
As artificial intelligence systems become integral to decision-making, ensuring fairness and transparency in AI models has become a critical priority. Explainable AI (XAI) techniques have emerged to enhance interpretability, enabling users to understand how machine learning models reach conclusions. Organizations now document model architectures, training data sources, and decision-making processes to maintain transparency. AI governance frameworks emphasize rigorous testing and validation protocols, ensuring models function equitably across diverse demographic groups.
Bias Detection and Mitigation Strategies
One of the most significant challenges in AI development is mitigating bias in machine learning algorithms. Organizations are deploying sophisticated bias detection frameworks that analyze decision-making across multiple demographic factors. This multi-layered approach ensures that AI models do not disproportionately impact specific groups. By employing intersectional bias analysis, organizations can reduce discriminatory outcomes while maintaining model accuracy. Performance monitoring systems track fairness metrics and environmental impact, ensuring ethical AI deployment.
Strengthening Compliance with Data Regulations
In an era of stringent privacy laws, organizations must comply with regulations such as GDPR and the California Consumer Privacy Act (CCPA). To navigate these complex frameworks, companies are implementing model-based compliance verification techniques. Automated compliance checks and detailed data processing maps provide a structured approach to regulatory adherence. Dynamic consent management systems empower users with control over their personal data, allowing for real-time adjustments to privacy preferences.
Governance and Oversight in Data Protection
Modern data governance frameworks integrate continuous monitoring systems that provide real-time insights into data access and policy compliance. Organizations now implement dynamic policy enforcement mechanisms that adapt based on risk assessment models. By leveraging federated governance structures, companies can balance centralized oversight with operational flexibility. Training and awareness programs play a crucial role in equipping teams with the knowledge necessary to maintain privacy-first systems. Advanced automation tools streamline compliance workflows while artificial intelligence helps identify potential security vulnerabilities. This integrated approach ensures robust data protection while enabling innovation through secure data sharing and collaborative analytics across organizational boundaries.
Future-Proofing Security with Advanced Architectures
The rapid evolution of cyber threats has necessitated the adoption of containerized security architectures. Organizations utilizing containerized monitoring solutions experience significant improvements in incident response times and resource utilization. Distributed logging frameworks, real-time threat analytics, and automated response systems are now fundamental components of modern security infrastructures. These technologies enhance resilience, ensuring organizations remain agile in the face of emerging threats.
The implementation of privacy-first architectures marks a paradigm shift in data processing and AI systems. By embedding security and ethical considerations at the core of technological development, organizations can build trust while remaining compliant with regulatory requirements. Amber Chowdhary’s insights underscore the necessity of integrating robust privacy mechanisms into AI and data infrastructure. Moving forward, businesses must continue to innovate while upholding ethical standards to ensure sustainable, privacy-conscious technological advancement.
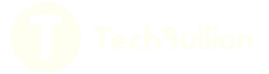