In today’s rapidly evolving healthcare landscape, managing patient risks and optimizing outcomes is crucial. Abrar Ahmed Syed explores how artificial intelligence (AI) is transforming healthcare through advanced risk stratification and early detection. By leveraging AI-driven technologies, healthcare organizations are improving their ability to predict and manage patient outcomes, making healthcare delivery more proactive and personalized. The integration of AI allows healthcare providers to act faster and more effectively, enhancing the overall quality of care. As a result, healthcare providers can offer more tailored treatments, ensuring better results and a more efficient healthcare system overall.
The Importance of Early Risk Detection
The shift toward preventive healthcare is becoming more evident as systems struggle with increasingly complex patient care management. AI helps healthcare organizations identify high-risk patients before critical conditions arise, improving patient outcomes and reducing hospital readmissions. Predictive models enable healthcare providers to prioritize patients most in need of attention, reducing costs and enhancing care delivery across the board. This proactive approach not only improves patient health but also contributes to better resource allocation within healthcare systems. By identifying risks early, healthcare providers can intervene sooner, preventing complications and improving overall efficiency.
AI Technologies Powering Risk Stratification
AI’s potential in risk stratification is driven by machine learning and natural language processing (NLP). Machine learning algorithms analyze vast amounts of clinical data to predict disease progression with high accuracy. These systems identify risk factors in real time, enabling informed decision-making. NLP models process unstructured medical text, extracting risk factors that improve diagnosis and care. As the models continue to learn, their predictions become more refined and dependable, offering deeper insights into patient health. This continuous learning process allows AI systems to adapt to new data, improving their ability to handle emerging health trends and unforeseen complications.
Optimizing Patient Outcomes with Personalized Care
AI-driven risk stratification is helping healthcare providers deliver personalized care. Predictive models enable better treatment plans tailored to individual risk profiles. This results in more efficient resource utilization and faster recovery times. By focusing on individual patient needs, healthcare providers can reduce unnecessary treatments and improve overall patient satisfaction, ultimately leading to better long-term health outcomes. Furthermore, AI helps to track patient progress over time, allowing providers to adjust care plans dynamically based on real-time data and emerging health conditions.
Overcoming Challenges in AI Integration
Integrating AI into existing healthcare systems presents challenges such as data interoperability and clinical workflow adaptation. He discusses the importance of ensuring AI transparency and aligning its decisions with medical best practices for successful adoption. Overcoming these challenges requires collaboration between clinicians and technologists to ensure that AI models support and enhance, rather than replace, human decision-making. This collaborative approach fosters trust in AI systems, ensuring that healthcare providers can make informed, data-driven decisions while maintaining the human element in patient care.
Security and Ethical Considerations in Healthcare AI
As healthcare data grows, ensuring its security and privacy remains a top priority. AI systems must include robust security features like encryption and role-based access controls to maintain confidentiality. Ethical concerns around AI bias are also addressed through diverse, fair data sets and continuous model testing. As AI systems evolve, maintaining transparency and accountability in decision-making will be crucial to building trust among patients and healthcare providers.
Future Directions: Emerging Technologies and the Future of Healthcare Analytics
The future of healthcare analytics will be driven by technologies like blockchain and edge computing. Blockchain ensures secure data sharing, and edge computing enables faster decision-making by processing data closer to the source, such as wearable devices. These technologies are expected to further enhance real-time patient monitoring and data sharing, making healthcare systems more agile, connected, and responsive to patient needs.
In conclusion, Abrar Ahmed Syed’s exploration of AI-driven risk stratification in healthcare highlights AI’s potential to optimize patient outcomes. By leveraging advanced AI, machine learning, and blockchain, healthcare organizations can improve patient care and operational efficiency. As AI continues to evolve, its role in healthcare will become even more essential for enhancing the quality of care, and healthcare systems will be better equipped to meet future challenges.
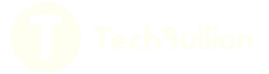