The field of insurance risk management is undergoing a major transformation, thanks to advancements in data analytics. Rakesh Maltumkar, an industry expert, explores how machine learning, natural language processing (NLP), and predictive modeling are reshaping the way insurers assess risk and serve their customers. These innovations are not only increasing efficiency but also reducing fraud and improving customer satisfaction.
Machine Learning: Enhancing Fraud Detection
Fraud detection has traditionally been a major challenge for insurers, but machine learning algorithms are proving to be game-changers. Advanced models like Random Forest and XGBoost have improved fraud detection accuracy rates to over 95%, significantly outperforming traditional statistical methods. These AI-powered systems analyze vast datasets, identifying subtle patterns indicative of fraudulent claims. Furthermore, real-time fraud detection has reduced false positives from 23% to just 7.8%, saving insurers millions of dollars annually.
NLP: Automating Claims Processing
Natural Language Processing (NLP) is streamlining claims processing by extracting essential information from unstructured documents with 88.7% accuracy. Computer vision technology further enhances this by analyzing accident photos and damage assessments with over 94% accuracy. These innovations are significantly reducing processing times and enhancing decision-making, leading to an improved customer experience. In fact, AI-powered claim analysis has cut processing times by 71.3%, allowing insurers to handle applications in minutes rather than days.
Predictive Modeling: Accurate Risk Assessment
Predictive modeling is revolutionizing how insurers assess risk. Advanced analytics tools now incorporate external data sources such as real-time weather updates, IoT sensor information, and demographic trends to create dynamic risk profiles. This has increased risk prediction accuracy to 83.5%, allowing insurers to implement early intervention strategies and reduce preventable losses by over 40%.
Data Integration and Real-Time Processing
One of the biggest challenges insurers face is managing vast amounts of data from diverse sources. Advanced ETL pipelines using AI-driven transformations now ensure data consistency with 94.8% accuracy. In-memory computing and adaptive load balancing have optimized system performance, reducing processing latencies to under 200 milliseconds while handling thousands of concurrent requests. These improvements allow insurers to operate with greater agility and efficiency.
AI-Driven Personalization and Customer Insights
AI-powered customer segmentation is redefining insurance personalization. Machine learning algorithms classify customers into distinct risk categories with over 83.9% accuracy, allowing insurers to offer tailored policies. Sentiment analysis tools leveraging NLP can detect customer dissatisfaction with 78.9% precision, enabling proactive interventions that boost retention rates. AI-based risk profiling further refines pricing strategies.
The Role of IoT in Risk Management
The integration of IoT devices is enhancing real-time risk monitoring. Smart sensors in homes and vehicles collect vast amounts of data, enabling insurers to adjust premiums dynamically based on actual risk exposure. These IoT-driven insights have reduced claim frequencies by 32.4% in property insurance and improved predictive modeling accuracy by nearly 38%. Furthermore, edge computing solutions ensure rapid processing of telemetric data, reducing latency by 88.5%.
Scalability and Automation in Insurance Operations
Cloud-based distributed computing is enabling insurers to scale their operations effortlessly. AI-driven automation has reduced manual intervention by nearly 70%, while real-time monitoring tools detect system inefficiencies with over 92% accuracy. These improvements are optimizing infrastructure costs and ensuring seamless claim handling, even during peak periods such as policy renewal seasons.
Future Innovations: Reinforcement Learning and Smart Infrastructure
Emerging technologies such as reinforcement learning and federated AI systems are poised to take insurance risk management to the next level. These advancements will refine premium optimization strategies and improve risk assessment models while maintaining privacy compliance. Additionally, the adoption of smart infrastructure, including connected urban sensors, is set to revolutionize location-based risk assessments, improving accuracy by nearly 40%.
In conclusion, Advanced data analytics is transforming the insurance industry, enhancing fraud detection, claims processing, and risk assessment. With technologies like machine learning, NLP, and IoT integration, insurers can offer more precise and efficient services while reducing operational costs. As the industry continues to embrace AI-driven solutions, it is evident that the future of insurance will be increasingly automated, personalized, and data-driven. Rakesh Maltumkar emphasizes that embracing these innovations will be key to staying competitive in an evolving landscape.
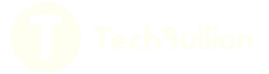