The rapid evolution of financial technology has unlocked unprecedented opportunities, but it has also introduced complex security challenges. As digital transactions surge globally, the risks of fraud have intensified, demanding innovative solutions. Preethi Ravisankar, an expert in artificial intelligence and financial security, explores how AI-powered fraud detection systems are transforming risk management in the fintech sector. Her insights into machine learning’s role in combating financial fraud provide a compelling look at the future of digital security.
Machine Learning: A Game-Changer in Fraud Detection
The integration of machine learning has revolutionized fraud detection by enabling financial institutions to analyze vast volumes of transaction data in real-time. Unlike traditional rule-based fraud detection methods, machine learning algorithms continuously adapt to emerging threats. These systems process millions of transactions per second, achieving high accuracy while minimizing false positives.
Supervised and Unsupervised Learning in Risk Management
Machine learning employs two primary approaches to fraud detection: supervised and unsupervised learning. Supervised learning relies on historical labeled transaction data to identify known fraud patterns. It excels in recognizing subtle correlations that human analysts might overlook. Meanwhile, unsupervised learning is crucial for detecting new fraud schemes by identifying anomalies in transactional behavior. Advanced algorithms continuously adapt to evolving threats in real-time financial monitoring systems.
Real-Time Monitoring for Enhanced Security
Modern fraud detection systems leverage real-time transaction monitoring to identify suspicious activities instantaneously. These systems analyze multiple transaction attributes, including purchase locations, device fingerprints, and spending habits. Advanced algorithms detect anomalies within milliseconds, ensuring potential threats are flagged before any financial damage occurs. For instance, a sudden high-value purchase from an unusual location might trigger an alert, prompting additional security checks.
Behavioral Analytics: A Personalized Security Approach
Behavioral analytics has become a cornerstone of AI-driven fraud detection. By analyzing user behavior, including spending habits and device usage, AI can create a unique financial profile for each individual. When deviations occur—such as an abrupt increase in spending frequency or transactions in unfamiliar locations—these systems raise red flags. The continuous learning capabilities of these models ensure they evolve with users’ changing financial behaviors, minimizing unnecessary transaction blocks while maximizing fraud prevention.
Anomaly Detection: Identifying Hidden Threats
AI-driven fraud detection systems excel at identifying unusual transaction patterns that might indicate fraud. By analyzing various data points, including transaction frequency, merchant categories, and geographical locations, these systems can pinpoint irregularities that human analysts might miss. For example, a personal account suddenly engaging in high-value business transactions could trigger further scrutiny. This ability to detect subtle irregularities strengthens overall security frameworks.
Balancing Security and User Experience
One of the biggest challenges in fraud prevention is balancing security with a seamless user experience. Modern fraud detection systems implement risk-based authentication, dynamically adjusting security measures based on transaction risk levels. Routine low-risk transactions require minimal authentication, while high-risk activities prompt additional verification steps, such as biometric authentication or multi-factor authentication. This approach minimizes user frustration while maintaining robust security protocols.
Cross-Border Payment Security
With the rise of international digital transactions, ensuring security across multiple jurisdictions has become a priority. AI-powered fraud detection systems analyze cross-border payments in real-time, adapting to diverse regulatory environments. By monitoring transaction flows and identifying unusual routing patterns, these systems can detect suspicious activities linked to money laundering or cybercrime. The integration of geospatial anomaly detection further strengthens international fraud prevention efforts.
The Future of AI in Fraud Prevention
AI-powered fraud detection is continuously evolving, incorporating advancements such as deep learning, natural language processing, and predictive analytics. These innovations enhance fraud prevention capabilities by improving detection accuracy and reducing false positives. As AI-driven security measures become more sophisticated, financial institutions will be better equipped to combat fraud while ensuring a smooth customer experience.
In the conclusion ,Preethi Ravisankar highlights how AI and machine learning have reshaped fraud detection and risk management. The combination of real-time monitoring, behavioral analytics, and anomaly detection has significantly strengthened financial security. By continuously learning and adapting to emerging threats, AI-powered fraud prevention systems are setting new standards in digital security. As fintech continues to evolve, AI-driven fraud detection will remain a cornerstone of safeguarding financial transactions in an increasingly digital world.
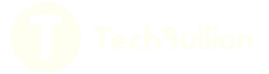