In the fast-evolving world of artificial intelligence and machine learning, the importance of rigorous quality assurance cannot be overstated. As AI technologies increasingly permeate industries like healthcare, voice assistants (like Amazon’s Alexa), and smart devices, ensuring their reliability and performance is critical. Pradeesh Ashokan, a Senior QA Engineer at Machinify and IEEE Senior Member, has spent the last six years mastering the complexities of testing AI/ML systems. From working with Siri co-founders at Viv Labs and Riva Health to developing FDA-compliant QA processes for medical devices, Ashokan is shaping the future of QA for cutting-edge technologies.
Revolutionizing QA for AI and ML Systems
AI/ML systems are fundamentally different from traditional software. They rely on probabilistic models and large datasets, which introduce unique challenges for testing. A recent article in The Wall Street Journal highlights efforts to rigorously test its models for vulnerabilities, such as hacking capabilities, to prevent potential misuse. This underscores the importance of thorough evaluation before deploying AI technologies. Ashokan, who played a pivotal role in scaling Viv Labs’ AI assistant (later Samsung’s Bixby) to millions of devices, explains the complexity: “Testing AI is not just about functional correctness. It’s about ensuring the models perform reliably under diverse real-world scenarios.”
At Viv Labs, Ashokan developed innovative validation techniques for natural language processing algorithms.His efforts improved regression test coverage from 50% to 80%, significantly enhancing the assistant’s accuracy and reliability across mobile, TV, and IoT devices.
“The biggest challenge in AI testing is variability,” Ashokan notes.
Pioneering QA in regulated industries
In highly regulated environments like healthcare, quality assurance is critical to ensuring compliance, safety, and trust. AI-driven healthcare solutions, such as ML-based cardiovascular health monitoring systems, must adhere to rigorous standards to deliver accurate and reliable outcomes.
“Testing in healthcare isn’t just about functionality; it’s about compliance, safety, and trust,” says Ashokan, who led QA efforts for Riva Health’s wireless blood pressure cuff. By implementing automated testing pipelines, he reduced regression testing time by 60% while improving coverage, helping the system meet strict regulatory standards.
Scaling QA for the Future of AI
As industries increasingly rely on AI/ML technologies, QA is evolving to meet new challenges. In healthcare, where AI models are used for critical decision-making, robust testing practices are essential to ensure accuracy and reliability. Creating complex test scenarios and automated test suites is becoming standard to validate ML algorithms effectively, with some achieving up to 95% accuracy in predicting treatment outcomes.
“The future of QA lies in automation and collaboration,” says Ashokan, who is a published author on Nanotechnology Perceptions and SARC, and focuses on advancing these practices.
A Vision for the QA Community
Beyond his technical contributions, Ashokan is a passionate advocate for knowledge-sharing and community building in the QA ecosystem. “QA isn’t just about finding bugs,” Ashokan who also is the recipient of the 2018 Individual Veev Cup award, emphasizes. “It’s about building trust—between developers, businesses, and the end users who rely on these technologies.”
As AI and ML continue to revolutionize industries, the importance of QA will only grow. Leaders like Pradeesh Ashokan are setting new benchmarks, ensuring these systems are not only functional but also reliable, compliant, and ready for real-world use.
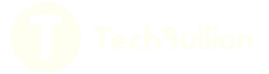