In an era driven by data, real-time analytics is redefining how businesses process information and make decisions. Dileep Kumar Siripurapu, an expert in the field, explores the technical foundations and emerging innovations in real-time data analytics, shedding light on its transformative impact. His insights offer a deep dive into cutting-edge technologies that are shaping the future of data-driven decision-making.
The Shift to Real-Time Processing
The rapid expansion of data streams has made real-time analytics an essential component of modern business strategies. Traditional batch processing methods are becoming obsolete as organizations demand immediate insights to remain competitive. Real-time analytics allows businesses to process vast volumes of data in milliseconds, enabling faster decision-making and enhanced operational efficiency.
Event-Driven Architecture: The Backbone of Real-Time Analytics
A key innovation in real-time analytics is event-driven architecture (EDA). Unlike conventional pull-based systems, EDAs leverage push-based models that significantly reduce network congestion and enhance system responsiveness. By decoupling system components, EDA facilitates seamless scalability, ensuring that organizations can efficiently process high-frequency data events with minimal latency.
Edge Computing: Reducing Latency and Improving Efficiency
The integration of edge computing with real-time analytics is a game-changer. Instead of relying on centralized cloud processing, edge computing enables data processing closer to its source. This minimizes latency, enhances response times, and reduces bandwidth costs. Organizations implementing edge analytics have reported significant improvements in operational efficiency and real-time decision-making.
Artificial Intelligence and Machine Learning at the Edge
AI and ML models are now being deployed at the edge, further amplifying the capabilities of real-time analytics. By reducing the need for continuous data transmission to centralized servers, edge AI enhances security, reduces computational overhead, and enables autonomous decision-making. These advancements are particularly beneficial in sectors such as healthcare, manufacturing, and financial services.
Quantum Computing: The Next Frontier
While still in its nascent stages, quantum computing presents immense potential for real-time analytics. Early implementations have demonstrated exponential improvements in processing speeds, enabling organizations to tackle complex data challenges with unprecedented efficiency. Quantum algorithms are poised to revolutionize fields such as fraud detection, predictive analytics, and optimization problems.
5G Networks: Accelerating Real-Time Data Processing
The deployment of 5G technology is another major enabler of real-time analytics. With ultra-fast data transmission speeds and minimal latency, 5G networks allow businesses to process real-time data at an unprecedented scale. This innovation is particularly impactful in industries that require instant data processing, such as autonomous vehicles, smart cities, and financial markets. The enhanced connectivity provided by 5G infrastructure enables organizations to collect and analyze massive amounts of sensor data in real-time, facilitating more accurate predictions and faster decision-making.
Performance Optimization and Data Quality
For real-time analytics to be effective, organizations must prioritize performance optimization and data quality management. Techniques such as intelligent partitioning, in-memory computing, and automated anomaly detection play a crucial role in ensuring data accuracy and system reliability. By implementing best practices in data governance, businesses can maximize the value of their analytics initiatives.
Ensuring Fault Tolerance and System Reliability
Real-time analytics systems must be resilient to failures. Advanced fault-tolerance mechanisms, including predictive fault detection and machine learning-driven recovery processes, ensure high availability and minimal downtime. Organizations investing in robust failover strategies and distributed architectures achieve near-perfect uptime, enhancing the reliability of their real-time analytics solutions.
Security Considerations in Real-Time Data Processing
With increased data velocity comes the challenge of securing sensitive information. Implementing strong encryption, access controls, and continuous security monitoring is essential to protecting real-time data. Organizations that prioritize cybersecurity measures can mitigate risks and ensure compliance with regulatory standards.
Future Outlook: The Continuous Evolution of Real-Time Analytics
The landscape of real-time analytics continues to evolve, driven by advancements in computing, AI, and connectivity. As businesses strive for greater efficiency, innovations such as federated learning, decentralized data processing, and advanced predictive models will further redefine the capabilities of real-time data analytics.
In Concuslion ,Dileep Kumar Siripurapu’s analysis underscores the profound impact of real-time analytics on industries worldwide. As organizations continue to embrace these innovations, the ability to process and act on data instantaneously will be a defining factor in their success.
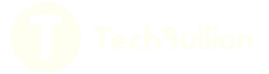