In this modern era of financial fraud detection, traditional rule-based systems are being outpaced by hybrid models that seamlessly integrate human expertise with data engineering frameworks. Highlighted in the work of Prakash Babu Sankuri, an expert in this field, these innovations have significantly enhanced detection accuracy, reduced false positives, and improved operational efficiency, enabling organizations to stay ahead of increasingly sophisticated fraud schemes.
The Rise of Human-Machine Collaboration
Modern fraud detection systems leverage the synergy between human insight and machine learning, creating a robust framework that outperforms standalone methods. By combining human analytical expertise with advanced algorithms, these systems can analyze complex transactional data and detect fraud patterns that traditional models would miss. Machine learning algorithms can achieve detection rates as high as 97% when paired with human contextual analysis, offering unprecedented accuracy and adaptability.
Real-Time Analytics and Transaction Monitoring
A cornerstone of contemporary fraud detection is the implementation of real-time analytics platforms. Capable of processing thousands of transactions per second, these systems flag suspicious activities within milliseconds. This rapid detection, enhanced by human oversight, has reduced false positives by up to 60%, enabling quicker response times and more accurate risk assessments. Such efficiency is vital in combating increasingly sophisticated fraud schemes, including synthetic identities and automated attacks.
Building a Strong Technical Foundation
Modern fraud detection systems rely on advanced technical infrastructure to manage massive volumes of financial data. These architectures often integrate data lakes capable of processing over 100 terabytes daily. By employing a combination of batch and stream processing, systems ensure seamless ingestion of structured, semi-structured, and unstructured data from diverse sources. Lambda architectures, which blend real-time and historical data processing, have further reduced latency, enhancing the timeliness and reliability of fraud detection efforts.
Optimizing Data Quality and Storage
Data quality and storage optimization are critical in maintaining the integrity of fraud detection models. Automated data validation frameworks achieve accuracy rates exceeding 99.9%, ensuring consistent performance even as data sources and volumes grow. Tiered storage solutions balance cost and performance by retaining critical data for immediate analysis while archiving historical data for model training and pattern analysis. This approach not only improves efficiency but also significantly reduces operational costs.
The Role of Collaboration Platforms
To maximize the effectiveness of fraud detection systems, cross-functional collaboration is essential. Platforms that facilitate real-time communication between data engineers and analysts have streamlined workflows and reduced decision-making times by up to 40%. By integrating knowledge-sharing tools, such as interactive dashboards and automated alerts, organizations can continuously refine detection models and respond swiftly to emerging threats.
Advanced Detection and Analysis Frameworks
Modern frameworks incorporate sophisticated analytics and structured methodologies to enhance fraud detection capabilities. From feature engineering that captures complex behavioral patterns to dynamic risk-scoring algorithms that prioritize alerts, these systems enable accurate and efficient analysis. Automated case routing and enrichment tools further reduce investigation times, allowing analysts to focus on higher-order tasks.
Challenges and Risk Management
Despite advancements, fraud detection systems face challenges such as data privacy, scalability, and regulatory compliance. Privacy-preserving machine learning techniques and cloud-based elastic infrastructure have addressed these issues, improving system availability and reducing costs. Additionally, strategies to minimize false positives, such as adaptive thresholding and continuous model retraining, have improved operational efficiency and customer experience.
The Path Forward
The future of fraud detection lies in further refining the collaboration between human expertise and technological innovation. Emerging technologies, such as deep learning and behavioral biometrics, promise to enhance detection capabilities and reduce fraud losses. Predictive analytics and industry-wide threat intelligence sharing will also play pivotal roles in proactively identifying and mitigating risks.
In conclusion, Prakash Babu Sankuri’s work underscores the transformative potential of integrating human expertise with data engineering in financial fraud detection. By embracing advanced analytics, real-time processing, and collaborative workflows, organizations can achieve unparalleled efficiency and accuracy in combating fraud. This approach not only strengthens financial systems but also sets a benchmark for innovation in data-driven risk management, paving the way for more proactive and resilient fraud prevention strategies. The ongoing evolution of these technologies ensures they remain adaptable to emerging threats and ever-changing regulatory landscapes.
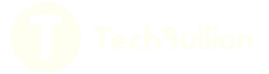