Artificial intelligence (AI) has become an integral part of modern enterprises, but data privacy concerns remain a major challenge. Sunil Kumar Gosai, an expert in AI-driven computing, explores an innovative solution in his latest work on federated learning (FL) in hybrid cloud environments. His research highlights how organizations can achieve high-performance machine learning while maintaining data sovereignty.
The Federated Learning Paradigm
Traditional AI models rely on centralized data storage, increasing risks to privacy and security. Federated learning transforms this approach by enabling decentralized training across multiple locations while keeping raw data private. This method enhances security, minimizes data breaches, and allows AI models to learn from diverse datasets. As a result, federated learning fosters robust, unbiased, and privacy-compliant AI systems without compromising performance or data integrity.
Enhancing Data Privacy and Security
A key advantage of federated learning (FL) is its ability to protect sensitive data. Organizations can train AI models locally without transferring private datasets to external servers, significantly reducing exposure to security threats. This approach minimizes data breaches and ensures compliance with strict privacy regulations. Studies show that FL implementations have resulted in a 43% decrease in privacy-related incidents, making it a powerful solution for secure and responsible AI development..
Hybrid Cloud: The Perfect Environment for FL
Hybrid cloud architectures, combining private and public cloud infrastructures, create an optimal environment for federated learning. By distributing workloads across multiple environments, organizations enhance security and performance. Research indicates that FL in hybrid cloud setups reduces cross-environment data transfers by 78.5%, significantly boosting efficiency. This approach ensures seamless AI training while maintaining data privacy and minimizing operational costs.
Optimized Communication and Model Aggregation
Successful federated learning (FL) relies on efficient communication and aggregation. Advanced compression techniques and secure aggregation protocols reduce bandwidth usage while preserving model accuracy. Experiments show compression ratios of up to 32:1, cutting network congestion and speeding up training. These optimizations enhance FL’s scalability, making it a practical solution for large-scale AI deployments.
Improved Model Performance with Adaptive Training
Federated learning (FL) ensures high model accuracy while operating in a decentralized manner. Organizations leveraging FL have reported up to a 28% accuracy improvement over traditional siloed training methods. Advanced techniques such as adaptive batching and differential privacy further enhance performance while maintaining stringent data protection. This seamless integration of accuracy and security makes FL a robust and reliable solution for modern AI applications across various industries.
Scalability and Efficiency Gains
Federated learning (FL) offers exceptional scalability, enabling organizations to train models across thousands of distributed nodes. Research indicates that modern FL deployments can support up to 15,000 concurrent nodes while sustaining over 92% training efficiency. Moreover, adaptive resource allocation has significantly reduced central computational demands by 87.3%, enhancing cloud infrastructure utilization. This efficiency makes FL a highly effective solution for large-scale AI applications while ensuring optimal resource management.
Addressing Challenges in Federated Learning
While FL offers significant advantages, it also faces challenges like communication overhead and managing non-uniform data distributions. Innovations in gradient sparsification and structured aggregation have addressed these issues, cutting communication costs by 85% and boosting model convergence rates. Future developments in post-quantum cryptography and edge-device optimizations are expected to enhance FL’s security and efficiency, making it even more effective for large-scale AI applications across diverse computing environments.
Future Prospects and Industry Adoption
As AI regulations tighten, federated learning (FL) is becoming a crucial solution for privacy-preserving machine learning. Sectors such as healthcare and finance are adopting FL to protect sensitive data while leveraging AI’s capabilities. Studies project a 456% increase in FL deployments by 2027, emphasizing its expanding role in ensuring data security while driving innovation across various industries and distributed computing environments.
In conclusion, federated learning marks a transformative shift in AI development, striking a balance between innovation and data privacy. As Sunil Kumar Gosai highlights, FL empowers collaborative intelligence while ensuring robust security, making it a vital technology for the future. With ongoing advancements, FL is poised to reshape AI’s role in enterprises and beyond, driving secure and efficient machine learning across various industries.
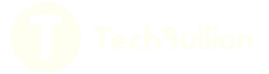