Gone are the days when individuals or businesses could formulate strategies without paying much attention to what was happening in the tech space. The reality is that we live in a tech-driven landscape and businesses that align themselves accordingly stand to achieve a competitive edge. Unless you live under a rock, you will agree that artificial intelligence (AI), is a term that dominates not only headlines but also boardrooms and product pitches.
Yet, amidst this flurry of attention, one question arises: does it matter what we call it? Think about it for a moment. The real debate isn’t whether it’s AI, machine learning (ML), or deep learning – these are distinct fields with clear differences. The real noise comes from the terms AI Automation, AI Systems, and AI Agents, which are often used interchangeably, despite representing different concepts.
Should businesses invest their energy in debating these definitions, or should they focus on what truly matters—solving problems and delivering results? While terminology can help provide clarity, getting caught up in these distinctions can distract from what AI is actually doing: enhancing efficiency, optimizing decision-making, and driving innovation.
Adrien Thomas, co-founder of Crescendo, AI Automation agency, and thought leader, has been vocal about the importance of shifting this focus. With strong experience in advising businesses on implementing and leveraging AI solutions, Adrien highlights that the fixation on terminology can detract from the core purpose of AI: making life and work more efficient and impactful. As Adrien succinctly puts it, “Clients don’t care what you call it, as long as it works. They care about time they’ll save, results they’ll get, and problems you’ll solve.” Shortly, we will explore why the emphasis should shift from semantics to solutions and how organizations can benefit by doing so.
The Debate Over AI Terminology
The discourse surrounding AI terminology often centers on distinctions between related but distinct concepts. The real debate isn’t about Artificial Intelligence (AI), Machine Learning (ML), or Deep Learning—each of these has clear definitions and roles in the AI ecosystem. Instead, the real noise in business discussions arises from the blurred lines between AI Automation, AI Systems, and AI Agents—terms that are frequently misunderstood or used interchangeably.
Consider this common scenario: A company executive asks, “Should we invest in AI Automation or AI Agents?” The question itself implies a false contrast. AI Automation focuses on rule-based tasks that require minimal human intervention. AI Systems integrate multiple AI capabilities to support decision-making and complex workflows. AI Agents go a step further by dynamically adapting to inputs, learning over time, and autonomously taking action. These are not competing concepts but different levels of AI implementation, each serving distinct business needs.
Adrien Thomas emphasizes that these debates can be counterproductive. Instead of uniting teams around a shared goal, they create silos and slow decision-making. “Arguing about whether it’s AI Automation or AI Agents doesn’t help your client solve their problem faster,” he notes. “What matters is the outcome.” By getting bogged down in terminology, businesses risk delaying or even derailing projects that could deliver tangible benefits.
The Real Priority: Delivering Solutions
At its core, AI should be about solving problems. Whether it’s streamlining operations, enhancing customer experiences, or uncovering new market opportunities, the goal of any AI implementation is to add value. This pragmatic approach requires a shift in focus from “what” the technology is to “how” it can be used effectively.
One of the key reasons businesses need to prioritize outcomes over definitions is that most stakeholders-including clients, end-users, and even many decision-makers-don’t care about the technical intricacies of AI. They care about results.
Adrien Thomas captures this sentiment perfectly: “Clients don’t care what you call it, as long as it works.” This statement underscores the need for businesses to align their AI initiatives with client expectations and tangible deliverables. Instead of worrying about whether it’s AI agent, AI systems, AI Automation, or another term, businesses should ask themselves questions like:
- What problem are we solving?
- How will this technology improve outcomes?
- How quickly can we deliver results?
By framing AI projects around these questions, organizations can focus their efforts on delivering value rather than getting lost in semantics.
Examples of Effective Solutions
The power of focusing on outcomes over terminology is evident in countless successful AI applications. Let’s consider the following examples:
- Customer Support Automation
Many businesses/companies have implemented chatbots to handle customer inquiries. While these tools are often labeled as AI-powered, the focus isn’t on the term “AI”-it’s on functionality. Whether the underlying technology involves natural language processing (NLP), ML, or basic rule-based algorithms, the goal is the same: to provide faster, more efficient customer support. Businesses that emphasize results – such as reduced wait times and improved customer satisfaction-achieve greater success than those fixated on the “correct” terminology.
- Predictive Maintenance in Manufacturing
In industrial settings, predictive maintenance systems use data to anticipate equipment failures before they occur. These systems rely on sophisticated algorithms, but whether they’re labeled as AI System or AI Automation is irrelevant to the factory managers who benefit from fewer breakdowns and increased uptime. The focus remains squarely on the solution’s impact.
- Personalized Marketing Campaigns
Retailers and e-commerce platforms use AI to deliver personalized recommendations to customers. Whether the solution is described as AI Agents, AI systems, or data analytics doesn’t matter to the end-user-what matters is the personalized experience and the increased likelihood of purchase. By prioritizing customer satisfaction and business outcomes, these companies successfully leverage technology without getting bogged down in terminology.
The aforementioned examples demonstrate that the value of AI lies in its ability to address real-world challenges. Businesses that concentrate on the “why” and “how” rather than the “what” are better positioned to achieve meaningful results.
Granted, it’s easy to get caught up in the semantics, especially in the fast-paced world of AI. However, as Adrien Thomas wisely points out, the debate over terminology often distracts from what truly matters-delivering solutions that work. By shifting the focus from definitions to outcomes, businesses can unlock the full potential of AI and create real value for their clients and stakeholders.
Ultimately, the goal of any AI initiative should be to solve problems, streamline operations, and improve lives. Whether it’s called AI automation, AI agents, or something else entirely, the label is secondary to the results. Organizations that adopt a solution-oriented mindset will not only avoid unnecessary debates but also position themselves as leaders in their fields.
So, the next time you’re faced with a conversation about AI terminology, take a step back and ask: What problem are we solving? The answer to that question is what really matters.
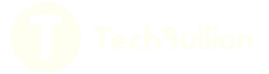