Cloud computing is continuously evolving, with one of the latest innovations being the development of advanced resource management for AI-driven platforms. In this article, Mouna Reddy Mekala explores the challenges and solutions in dynamically scaling resources for generative AI workloads, offering a glimpse into the future of cloud optimization.
Reimagining Resource Allocation for AI
Recent growth in generative AI has created unprecedented demand on cloud resources. Unlike traditional applications with predictable consumption, AI models, especially generative ones, face unique challenges due to fluctuating resource needs, making traditional scaling methods inadequate for rapid shifts
The article emphasizes that past approaches, relying on static resource allocation and reactive auto-scaling, result in inefficiencies, increased costs, and poor performance. During high-demand periods, traditional methods caused significant performance degradation, highlighting the need for dynamic, proactive scaling.
Introducing Dynamic Scaling: The Solution to Volatility
The proposed solution uses a dynamic resource management system combining predictive and reactive strategies. Machine learning forecasts AI workload needs, adjusts resources accordingly, reducing inefficiencies, avoiding over-provisioning, and minimizing latency.
Research shows the system achieves 87% resource utilization, improving efficiency. The predictive component processes real-time data to allocate resources for AI workloads, while the reactive component handles demand spikes for agile responses.
The Key to Success: Adaptive Scaling Logic
The centerpiece of this solution is its adaptive scaling logic, which continually adjusts to real-time conditions. Unlike traditional scaling models that rely on fixed thresholds, the adaptive system dynamically aligns resource availability with workload demands. Studies have shown that dynamic scaling can reduce resource wastage by as much as 67%, ensuring that resources are only used when necessary, thus optimizing cost-efficiency.
Additionally, the system incorporates sophisticated GPU allocation mechanisms that operate at a fine granularity of 0.25 GPU units. This precision significantly reduces over-provisioning, which is a common issue in traditional cloud platforms. By fine-tuning resource allocation to match real-time needs, the solution dramatically improves throughput, reducing function initialization times and increasing operational efficiency.
Platform-Specific Optimization for Greater Efficiency
The solution integrates platform-specific optimizations to enhance performance across various cloud environments like Databricks and Amazon EMR. Databricks’ Photon engine boosts query speeds by 7.4x, while EMR optimizations reduce instance provisioning latency by over 60%, demonstrating platform-aware scaling benefits.
This targeted approach ensures that AI workloads are not only managed efficiently but are also optimized for each specific platform’s capabilities. As a result, businesses can expect to see better overall performance, lower costs, and enhanced operational efficiency.
Continuous Improvement Through Predictive Maintenance
In addition to dynamic scaling, the system integrates predictive maintenance by monitoring performance metrics, identifying potential issues early, reducing downtime, and improving recovery times by over 70% through proactive maintenance.
Moreover, the ability to track resources with millisecond precision enhances the system’s responsiveness, particularly during peak demand. The framework’s continuous optimization ensures that it remains effective even as AI models grow more complex and demand increases.
Setting New Standards for AI Workload Management
The implications of this dynamic resource management solution extend far beyond just optimizing cloud resources. By enabling organizations to handle more complex AI models efficiently, this approach lays the groundwork for the next generation of AI applications, especially in edge AI and real-time processing.
Future developments in AI architectures, particularly those requiring heterogeneous computing resources, will benefit greatly from this system. As AI workloads continue to evolve, the need for adaptive, intelligent scaling solutions will only increase, making this innovation a critical advancement in the field of cloud computing.
In conclusion, Mouna Reddy Mekala’s work offers a transformative perspective on AI workload scaling. By addressing the unique challenges posed by generative AI workloads and combining predictive with reactive scaling, this dynamic resource management system provides a far more efficient and cost-effective approach than traditional methods. The platform-specific optimizations for environments like Databricks and Amazon EMR, along with the integration of predictive maintenance, further enhance its practical value. This research not only solves current cloud computing challenges but also sets the stage for future innovations in edge AI and real-time applications, revolutionizing how we think about resource management in AI deployments.
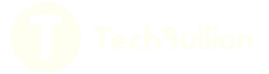