In this modern era telecommunication networks grow in complexity, ensuring reliability and minimizing downtime are crucial. Chandrasekhar Katasani explores an AI-powered predictive maintenance framework that leverages real-time streaming, cloud computing, and machine learning to prevent network failures. His work highlights innovations that improve operational efficiency, reduce disruptions, and optimize resource allocation. By integrating predictive analytics, telecom providers can detect issues before they escalate, enhancing overall network performance. These advancements not only reduce maintenance costs but also improve customer satisfaction by ensuring seamless connectivity.
The Need for Predictive Maintenance
Telecom networks depend on interconnected devices, and failures can cause service disruptions. Traditional reactive maintenance approaches lead to high costs and downtime. Predictive maintenance reduces outages by 50% and extends equipment lifespan by 40%. By analyzing real-time data, telecom operators detect failures early, improving network stability and efficiency. AI-driven analytics enhances fault detection accuracy, enabling proactive decision-making for infrastructure optimization.
AI-Powered Fault Detection
Real-Time Data Streaming
Modern predictive systems use real-time data streaming to process high-velocity telemetry from network devices. These platforms handle millions of data points per second, analyzing signal strength, power usage, and equipment temperature. Advanced streaming architectures ensure sub-millisecond latency for immediate anomaly detection.
AI-Enhanced Failure Prediction
Machine learning models trained on historical failure records can predict malfunctions with over 85% accuracy. Anomaly detection techniques improve fault detection precision, reducing false alarms while enabling proactive maintenance scheduling.
Scalable Cloud Infrastructure
Cloud-Based Computing for Large-Scale Processing
Cloud platforms store historical failure records for trend analysis. Serverless computing dynamically allocates resources, optimizing costs while ensuring high-speed processing. Cloud-based predictive maintenance allows telecom providers to analyze and manage vast amounts of network data efficiently.
Automated Data Preprocessing
High-quality data is key to predictive accuracy. Automated data preprocessing reduces anomalies by 60%, ensuring over 99.5% data accuracy. Advanced feature engineering techniques provide deep insights into network health and performance trends.
AI-Driven Fault Detection
Multi-Dimensional Anomaly Detection
AI-based anomaly detection systems analyze power fluctuations, temperature variations, and signal degradation to predict failures. These frameworks process large datasets and reduce false positives by 65%, improving fault detection.
Trend Analysis for Preventative Action
AI models analyze seasonal and long-term variations in network performance. Predictive analytics forecast maintenance needs in advance, reducing service interruptions.
AI Models for Network Health
Neural Networks for Fault Detection
Deep learning models, including convolutional neural networks (CNNs) and long short-term memory (LSTM) networks, analyze large volumes of network telemetry data. These models detect patterns that traditional methods miss, improving fault prediction accuracy by 35%.
Federated Learning for Data Privacy
Federated learning enables AI models to train on decentralized telecom data without transferring sensitive information, improving privacy and detection performance.
Operational Monitoring and Optimization
Intelligent Alerting and Prioritization
Predictive maintenance systems use AI-driven alert mechanisms to categorize failures based on urgency, improving response times and reducing unnecessary interventions.
Performance Dashboards for Real-Time Insights
Dynamic dashboards track network health metrics, providing visibility into device status, failure trends, and resource utilization. These insights help operators optimize maintenance schedules.
Business Impact
Cost Reduction and Efficiency Gains
Predictive maintenance reduces emergency repairs by 70%, lowering operational costs. Studies show improved maintenance efficiency by 60% and reduced equipment replacement costs by 30%.
Improved Service Quality
Proactive fault detection increases service availability to 99.99%, reducing customer-reported network issues by 55% and improving satisfaction.
Future of AI in Telecom
Edge AI for Real-Time Fault Detection
Edge AI processes data on devices, minimizing response times and enhancing network resilience.
AI-Driven Self-Healing Networks
Autonomous networks use AI to detect, diagnose, and resolve faults without human intervention, reducing downtime by 80%.
In conclusion, Chandrasekhar Katasani’s research highlights the transformative impact of AI-driven predictive maintenance in telecom networks. By leveraging real-time streaming, cloud computing, and machine learning, telecom operators can improve network reliability, reduce costs, and enhance service quality. As AI advances, predictive maintenance will remain essential to telecom infrastructure, ensuring seamless connectivity and operational efficiency. The integration of automated fault detection and self-healing networks will further revolutionize maintenance strategies, reducing manual intervention. These advancements will enable telecom providers to scale operations while maintaining high-performance standards in an increasingly digital world.
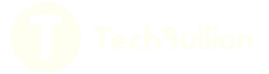