Artificial intelligence has steadily seeped into our everyday lives in subtle and not-so-subtle ways. Some examples are navigation tools, online shopping, facial recognition, and smart home devices. However, the average consumer may not realize that AI is playing an increasingly significant role in finance.
This article explores the rise of algorithmic trading, focusing on its promise and challenges as well as technological innovations that will drive performance and reliability in financial markets.
What Is Algorithmic Trading?
Currently, about 70 percent of trades on the U.S. stock market are initiated by AI-driven trading. Algorithmic trading (also known as automated trading, algo-trading, or black-box trading) uses machine learning to analyze data and follow a defined set of instructions — or algorithms — to place a trade.
This form of trading can outpace human traders in speed and accuracy. It also takes away factors such as human emotions that can come into play in the fast-paced and emotional roller-coaster of trading activities.
However, as many industries that have embraced AI have discovered, the use of AI in finance brings both advancements and important ethical and regulatory considerations. “I view AI as a tool for traders and investors,” says Harshit Khandelwal, CEO and co-founder of Pioneer Investing Inc., a fintech startup working to transform copy trading and stock trading through technology and data-driven insights.
“Professional and individual investors can harness AI in many ways, including selecting stocks, managing portfolios, and managing risk,” says Khandelwal, who served for six years as a senior software engineer for Amazon and led the launch of multiple projects which have contributed to generating over $1 billion in annual revenue for Amazon Retail.
“When used as part of the traders’ toolbox, algorithmic trading leveraging technology can democratize financial markets, empowering individuals to achieve their investment goals with confidence and ease.”
How Does Algorithmic Trading Work?
This form of AI follows a pre-determined set of rules to make trading decisions. The algorithm analyzes historical data to select and monitor assets tailored to the trader’s goals and risk tolerance. It can process data more rapidly and with more accuracy and precision than human traders, and it can do so at all hours.
For example, an investor using algorithmic trading no longer must monitor live stock prices to place an order. The system will monitor the price and moving average indicators of a stock to identify and execute a trading opportunity automatically.
“Many people new to this technology understandably are concerned with risk management,” says Khandelwal. “However, we Integrate real-time checks for margin limits, position sizing, and risk exposure into the system.”
Khandelwal lists the three core components of a trading engine.
- Market Data Processing System — handles real-time data streams and ensures low latency.
- Order Management System (OMS) — manages design considerations for trade execution, order placement, and cancellations.
- Risk Management System — integrates real-time checks for margin limits, position sizing, and risk exposure.
He adds that back-testing can be added to the system. This component harnessed available historical and real-time data to determine a successful and viable trading strategy.
What About Ethical Considerations?
Along with the significant advantages of algorithmic trading come critical ethical questions regarding fairness, transparency, and market stability.
Khandelwal stresses the importance of compliance with financial regulations to prevent unfair practices and market manipulation.
The Security Exchange Commission (SEC) and the Commodity Futures Trading Commission (CFTC) oversee algorithmic trading in the United States. Some of the SEC rules for algorithmic traders include:
- Registration and completion of education requirements with the Financial Industry Regulatory Authority (FINRA).
- Regular reports of trading activities, including strategies, order types, and execution times.
- Implementing controls to monitor market risk, credit risk, and conflicts of interest.
What’s Ahead for Algorithmic Trading?
As computer trading models continue to improve in the way they use data to predict market trends, they will become more and more sophisticated.
“With new advancements in deep learning and natural language processing, these trading systems will be able to better understand broader market dynamics and adapt to new data – including real-time news events — without any human intervention,” says Khandelwal.
He explains that machine learning already can be harnessed for predictive analytics, anomaly detection, and strategy development. “A few of the emerging trends I see in trading technology include copy trading, social trading, decentralized finance (DeFi), and blockchain-based smart contracts for trade settlements.”
“We expect quantum computing will become integrated into algorithmic trading systems, enabling them to compute complex pricing models, thereby greatly improving the accuracy of pricing in volatile markets.”
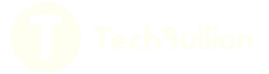