In the rapidly growing field of digital healthcare, leveraging artificial intelligence to streamline processes is becoming increasingly vital. Balamurugan Sivakolunthu Vel, a researcher in medical informatics, has developed a comprehensive BERT-based framework for automating medical document processing. His innovative approach focuses on tackling the unique challenges of healthcare documentation while improving efficiency, accuracy, and compliance.
The Healthcare Documentation Challenge
Healthcare systems manage vast amounts of data, with Electronic Health Records (EHR) accounting for over 44 billion terabytes globally. This enormous data volume, coupled with the complexity of medical terminology, creates significant bottlenecks in processing clinical notes and discharge summaries. Automating these processes with advanced language models offers an effective solution to improve efficiency and accuracy.
The Potential of BERT in Healthcare NLP
Transformer-based architectures like BERT (Bidirectional Encoder Representations from Transformers) have demonstrated remarkable potential in medical natural language processing (NLP). With accuracy rates of up to 89.7% in medical entity recognition tasks, BERT provides a solid foundation for improving documentation workflows. The proposed framework by Balamurugan incorporates domain-specific optimizations to enhance BERT’s performance in handling medical text.
Key Innovations in the Framework
Hierarchical Attention Mechanisms
The framework integrates a hierarchical attention mechanism, enabling the model to process data at multiple levels—document, section, and entity. This design enhances contextual understanding, which is critical for accurate classification and identification in complex medical documentation.
Distributed Computing for Scalability
The system leverages distributed training pipelines across edge computing nodes, achieving significant efficiency improvements compared to traditional centralized models. By incorporating dynamic load balancing, it ensures seamless scalability and consistent quality of service, even when processing millions of clinical documents annually.
Medical Vocabulary Injection
A dynamic medical vocabulary injection system ensures high accuracy in terminology recognition by maintaining an updated dictionary of medical terms. This component reduces false positives and improves classification precision, making it particularly effective in specialized healthcare domains.
Improving Workflow Efficiency and Accuracy
The framework’s design brings transformative benefits to healthcare organizations. Real-time processing reduces document handling times, improving operational efficiency and enabling faster decision-making. Tests conducted on adverse drug reaction reports demonstrated a precision rate of 92% and a recall rate of 90%. Additionally, the framework reduces manual data entry, minimizing administrative burdens and allowing healthcare professionals to focus more on patient care.
Real-World Applications
Adverse Drug Reaction Monitoring
The framework streamlines the processing of ADR reports, achieving near-human performance in causality and severity classification. This ensures timely identification of drug-related risks, enhancing patient safety.
Clinical Documentation
The system excels in processing clinical notes and discharge summaries, maintaining consistent accuracy across diverse document types. Its ability to integrate seamlessly with existing EHR systems makes it a practical solution for improving documentation workflows.
Decision Support Systems
With high precision in medical entity recognition, the framework supports clinical decision-making by extracting relevant data from complex documents. This capability is particularly valuable in multidisciplinary healthcare environments.
Addressing Implementation Challenges
Data Privacy and Security
Handling sensitive patient information requires robust security measures. The framework employs advanced encryption techniques, access control protocols, and compliance monitoring to ensure data integrity and privacy.
Integration with Legacy Systems
Integrating advanced solutions with existing healthcare infrastructures can be challenging. The proposed hybrid edge-cloud architecture facilitates smoother integration by combining resource optimization with automated load distribution.
Computational Demands
The system addresses high computational demands through efficient resource utilization, reducing processing bottlenecks and achieving fault tolerance rates above 95%. Smart scheduling algorithms further enhance operational reliability.
Future Directions
The framework opens the door to future enhancements in healthcare NLP. Potential advancements include integrating AI-driven predictive analytics to optimize resource allocation and exploring blockchain technologies for secure, transparent data sharing. These innovations would further improve system reliability and scalability, making healthcare documentation even more efficient.
In conclusion, Balamurugan Sivakolunthu Vel’s BERT-based framework represents a significant step forward in automating healthcare documentation. By addressing the unique challenges of the medical domain, this solution enhances accuracy and efficiency. As healthcare organizations generate increasing volumes of digital data, adopting such robust frameworks will be essential to improving clinical workflows and patient care. This innovation sets the stage for future advancements in medical NLP, contributing to a more effective healthcare system.
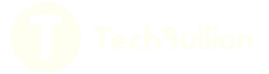