In this digital era, artificial intelligence continues to push boundaries, with multimodal AI representing a transformative leap in the field. This article delves into the latest innovations in this technology, drawing insights from expert Bhabani Sankar Nayak. His work explores these intelligent systems’ architectural evolution, performance enhancements, and future potential, shedding light on their growing impact across various domains.
Integrating Multiple Modalities for Enhanced Intelligence
Multimodal AI systems are designed to process and integrate diverse data sources, including text, images, speech, and sensory inputs, much like human cognition. Recent breakthroughs in these systems have demonstrated a 32.4% improvement in cross-modal learning tasks, surpassing single-modality models. Advanced vision-language frameworks have exhibited 89.7% precision in cross-modal alignment, ensuring superior comprehension and task execution. These capabilities have proven instrumental in applications requiring real-time decision-making, particularly in industrial automation and healthcare analytics.
A Layered Approach to Multimodal AI Architecture
The architecture of multimodal AI is structured into three fundamental layers: modal-specific processors, cross-modal integration, and a unified representation system. The first layer optimizes data processing from different modalities, leveraging innovations such as Vision Transformer architectures, which achieve a 96.2% accuracy rate in object detection tasks with reduced computational overhead. Meanwhile, natural language processing units have evolved to handle complex linguistic structures, processing up to 2048 tokens per batch with an accuracy of 92.4%.
At the integration level, attention-based mechanisms facilitate cross-modal feature correlation with 83.7% accuracy, operating at an optimized computational budget of 157 GFLOPS. Feature fusion networks enhance efficiency by preserving 94.1% of input information while reducing dimensionality. These advancements enable multimodal AI systems to process diverse inputs seamlessly, ensuring robust performance across various domains.
Tackling the Synchronization Challenge
One of the significant challenges in multimodal AI is data synchronization. Effective temporal integration must occur within a 32-45ms window to maintain optimal coherence. Delays beyond this range result in performance degradation of over 31%. Innovations in adaptive temporal mapping techniques have improved synchronization accuracy to 93.8%, even in variable network conditions. Additionally, neural-inspired architectures now achieve 91.6% accuracy in cross-modal correlation detection, enabling systems to process semantically related content rapidly.
Optimizing Resource Management and Computational Efficiency
Efficiency remains a crucial focus in multimodal AI development. Recent advancements in distributed processing architectures have improved throughput by 4.2x compared to traditional methods. Cross-modal attention mechanisms now compute at speeds of 3.2 TFLOPS while maintaining 86.7% memory bandwidth efficiency. Additionally, memory fragmentation has been reduced by 82.4% through optimized garbage collection mechanisms, allowing systems to support up to 32 concurrent processing streams while preserving system coherence.
Real-World Applications: Virtual Assistants and Healthcare Analytics
Multimodal AI is transforming industries, particularly virtual assistants and healthcare analytics. Virtual assistants now achieve 95.8% task completion rates, processing complex queries in 142ms, with speech recognition Word Error Rates reduced to 3.2% and real-time translation spanning 37 languages. In healthcare, diagnostic accuracy has improved by 32.8%, powered by advanced medical imaging processors handling 156 images per second with 99.9% resolution fidelity. Additionally, patient record parsing systems achieve 97.2% accuracy, ensuring precise medical data processing and decision-making.
The Future of Multimodal AI: Towards Human-Like Cognition
As multimodal AI evolves, future architectures will feature adaptive neural networks with 97.3% feature correlation accuracy, reducing processing latency to 15.7ms while preserving over 95.8% of cross-modal data. Innovations include haptic feedback, real-time environmental sensing, and biochemical signal processing. Experimental systems already process biochemical signals with 93.4% accuracy in real time. These advancements will revolutionize industries, enabling AI to interact seamlessly with the physical world, bridging the gap between human and machine intelligence.
In conclusion, Bhabani Sankar Nayak’s insights underscore the rapid evolution of multimodal AI, which is driving significant advancements across various applications, from virtual assistants to healthcare diagnostics. Through enhanced architecture, efficient data synchronization, and optimized resource management, these intelligent systems are redefining standards in artificial intelligence. As research progresses, multimodal AI will continue to bridge the gap between human and machine intelligence, unlocking innovative solutions to complex challenges and shaping the future of AI-driven technologies.
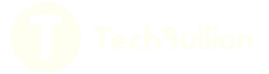