In today’s fast-paced and data-driven world, organizations are faced with increasingly complex decisions that require precision, speed, and adaptability. From supply chain optimization to personalized customer experiences, the stakes are high, and traditional decision-making methods often fall short. Enter artificial intelligence (AI)-driven software—an innovative solution designed to automate and enhance decision-making processes across industries. This blog post explores how AI-powered tools are transforming decision-making, the benefits they bring, challenges to consider, and real-world applications.
The Evolution of Decision-Making in Business
Historically, business decisions were made by humans relying on experience, intuition, and limited datasets. While these approaches worked well in simpler times, modern enterprises operate in environments characterized by vast amounts of structured and unstructured data, dynamic market conditions, and heightened competition. For instance, if you were to ask a question like how many restaurants in America, the sheer volume of data required to answer this—ranging from local directories to real-time market trends—highlights the complexity businesses face today. Human cognition alone is no longer sufficient to process this complexity efficiently.
Enterprises have turned to advanced analytics and machine learning models as a way to augment human decision-making capabilities. However, even these early solutions had limitations—they required significant manual input, lacked scalability, and struggled to adapt to rapidly changing scenarios. The next logical step was automating not just parts of the process but entire workflows through AI-driven software.
AI-driven software takes decision-making to the next level by leveraging algorithms capable of analyzing massive datasets, identifying patterns, predicting outcomes, and recommending—or even executing—actions autonomously. These systems can handle tasks ranging from routine operational choices to highly nuanced strategic decisions.
How AI Automates Complex Decisions
AI-driven software automates decision-making using several key technologies:
- Machine Learning (ML): ML algorithms learn from historical data to identify trends and make predictions. For example, predictive maintenance systems use ML to anticipate equipment failures before they occur, enabling proactive repairs.
- Natural Language Processing (NLP): NLP allows AI systems to understand and generate human language, making it possible to extract insights from text-based sources like emails, social media posts, or legal documents.
- Optimization Algorithms: These algorithms evaluate multiple variables simultaneously to find the best course of action under given constraints. For instance, logistics companies use optimization algorithms to determine the most efficient delivery routes.
- Rule-Based Systems: Some AI systems combine predefined rules with learned behaviors to ensure compliance with regulations while adapting to new information.
- Reinforcement Learning: This technique enables AI to improve its performance over time by experimenting with different strategies and learning from feedback loops. It’s particularly useful in dynamic environments such as financial trading or autonomous vehicles.
By integrating these technologies, AI-driven software creates a robust framework for handling complex decisions without constant human intervention.
Benefits of AI-Driven Decision Automation
The adoption of AI-driven software offers numerous advantages for businesses seeking to streamline their operations and stay competitive:
1. Speed and Efficiency
One of the primary benefits of AI is its ability to analyze large volumes of data quickly. What might take humans days or weeks to accomplish can be done in seconds or minutes by an AI system. This rapid processing capability ensures timely responses to critical issues, whether it’s detecting fraud in real-time or adjusting inventory levels based on demand forecasts.
2. Improved Accuracy
Human error is inevitable, especially when dealing with repetitive or intricate tasks. AI reduces the likelihood of mistakes by applying consistent logic and eliminating biases inherent in manual decision-making. For example, credit scoring models powered by AI provide more accurate assessments than traditional methods because they consider a broader range of factors.
3. Scalability
Unlike human teams, which require additional resources to scale up, AI systems can easily expand their capacity to handle increased workloads. Whether you’re managing millions of transactions per day or monitoring thousands of IoT devices, AI scales effortlessly.
4. Cost Savings
Automation leads to cost reductions by minimizing labor-intensive processes and optimizing resource allocation. For instance, chatbots powered by NLP reduce the need for customer service representatives, while predictive analytics helps prevent costly downtime in manufacturing plants.
5. Enhanced Customer Experiences
AI-driven personalization engines analyze user behavior to deliver tailored recommendations, promotions, and content. Companies like Amazon and Netflix rely heavily on AI to create hyper-personalized experiences that drive customer satisfaction and loyalty.
Challenges and Considerations
While AI-driven software holds immense promise, implementing it comes with certain challenges:
1. Data Quality and Availability
AI systems depend on high-quality, diverse datasets to function effectively. Poor-quality or biased data can lead to inaccurate results and flawed decisions. Organizations must invest in data governance practices to ensure their datasets are clean, relevant, and representative.
2. Ethical Concerns
AI raises ethical questions around transparency, accountability, and fairness. If an AI system makes a wrong decision, who is responsible? How do we prevent algorithmic bias from perpetuating discrimination? Addressing these concerns requires careful design and oversight.
3. Integration Complexity
Integrating AI into existing IT infrastructures can be challenging, especially for legacy systems. Businesses may need to overhaul their technology stacks or hire specialized talent to implement and maintain AI solutions successfully.
4. Resistance to Change
Employees may resist adopting AI-driven tools due to fear of job displacement or lack of understanding. Effective change management strategies, including training programs and clear communication about AI’s role, are essential to overcoming this barrier.
Real-World Applications of AI-Driven Decision Automation
AI-driven software is already being used across various sectors to tackle complex problems:
1. Healthcare
AI assists doctors in diagnosing diseases, recommending treatments, and predicting patient outcomes. For example, IBM Watson Health analyzes medical records and research papers to suggest personalized cancer therapies.
2. Finance
Banks and fintech firms leverage AI to detect fraudulent activities, assess loan eligibility, and optimize investment portfolios. Robo-advisors like Betterment use AI to manage clients’ assets automatically.
3. Retail
E-commerce platforms employ AI to forecast demand, manage inventory, and personalize shopping experiences. Walmart uses AI to optimize shelf stocking and pricing strategies.
4. Manufacturing
Smart factories utilize AI for quality control, production scheduling, and predictive maintenance. General Electric’s Predix platform monitors industrial equipment to minimize disruptions.
5. Transportation
Autonomous vehicles rely on AI to navigate roads safely, while ride-sharing apps like Uber use AI to match drivers with passengers and calculate fares dynamically.
The Future of AI-Driven Decision Automation
As AI technology continues to evolve, we can expect even greater advancements in decision automation. Emerging trends include:
- Explainable AI (XAI): Efforts to make AI systems more transparent so users can understand how decisions are made.
- Edge AI: Deploying AI directly on devices at the network edge to enable faster, localized decision-making.
- Collaborative AI: Systems that work alongside humans rather than replacing them, enhancing collective intelligence.
These innovations will further blur the lines between human and machine collaboration, paving the way for smarter, more resilient organizations.
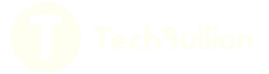