In this modern era, where artificial intelligence is reshaping industries, healthcare is witnessing a profound transformation through the integration of Large Language Models (LLMs). Madhu Babu Kola, in his extensive research, explores the role of these models in enhancing clinical decision support systems. His work highlights how the synergy between human expertise and AI is redefining diagnostics, improving treatment outcomes, and addressing long-standing challenges in healthcare delivery. These advancements enable personalized, data-driven, and efficient patient care.
The Evolution of Healthcare Diagnostics
Healthcare diagnostics have evolved from experiential practices to evidence-based methodologies, driven by advancements in technology. Traditional diagnostic approaches, while foundational, face challenges in managing the exponential growth of medical data. The integration of AI, particularly LLMs, provides clinicians with tools to process vast datasets and uncover patterns imperceptible to human analysis. This evolution addresses the need for rapid, accurate, and consistent diagnoses in increasingly complex healthcare environments. Additionally, it bridges gaps in expertise, ensuring comprehensive diagnostic insights across diverse medical domains.
The Role of AI in Healthcare Transformation
Artificial intelligence is revolutionizing healthcare by enabling the analysis of diverse medical data in real-time. LLMs and other AI technologies process clinical notes, imaging data, and genomic information, providing actionable insights that enhance clinical workflows. By integrating seamlessly with Electronic Health Records (EHR) and diagnostic systems, AI ensures that patient care is both data-driven and patient-centric. These technologies empower clinicians to make informed decisions swiftly while reducing diagnostic errors and improving overall treatment efficiency.
AI-Augmented Diagnostics: A New Standard
AI-driven diagnostic systems have set a new standard in medical imaging and clinical decision-making. These systems analyze X-rays, MRIs, and CT scans with unprecedented accuracy, detecting anomalies and supporting early diagnosis. Advanced LLMs integrate patient histories, lab results, and clinical guidelines to offer evidence-based recommendations. By improving diagnostic accuracy and reducing variability, these systems elevate the overall quality of care while ensuring timely interventions that can significantly improve patient outcomes.
Enhancing Treatment Planning with AI
AI’s impact extends beyond diagnostics to treatment optimization. LLMs analyze patient-specific data, including genetic profiles and medical histories, to personalize treatment plans. Predictive models evaluate treatment outcomes, minimizing risks and improving efficacy. This personalized approach ensures treatments align with individual patient needs and goals. By continuously learning from patient feedback and evolving clinical data, AI systems refine treatment strategies over time.
Data Security and Ethical Considerations
The integration of AI in healthcare demands robust ethical frameworks and data security measures. Modern AI systems employ advanced encryption, role-based access controls, and privacy-preserving computation to protect sensitive patient data. Ethical considerations include addressing algorithmic bias and ensuring fairness across diverse patient populations. By adhering to stringent regulatory standards, AI systems maintain trust while delivering equitable healthcare solutions. Transparent audit mechanisms and regular evaluations further ensure accountability and alignment with ethical guidelines.
Challenges in AI Implementation
Despite its potential, integrating AI into healthcare faces challenges such as infrastructure requirements, staff training, and cost management. Implementing AI systems requires seamless integration with existing workflows and continuous adaptation to evolving clinical needs. Comprehensive training programs for healthcare professionals ensure effective utilization of AI tools. Additionally, overcoming resistance to new technology through education and collaboration is critical to fostering trust and ensuring long-term success.
Future Prospects of AI in Healthcare
Emerging AI technologies, including federated learning and edge computing, promise to further enhance healthcare delivery. These advancements improve real-time data analysis, enabling faster and more accurate clinical decisions. As AI systems evolve, they are expected to play a pivotal role in population health management and patient engagement. Furthermore, advancements in AI transparency and explainability are likely to strengthen trust among clinicians and patients, paving the way for broader adoption.
In conclusion, Madhu Babu Kola’s research emphasizes the transformative potential of integrating Large Language Models into clinical decision support systems. By augmenting human expertise, AI-driven tools enhance diagnostic precision, optimize treatment strategies, and improve patient outcomes. This collaborative approach ensures that healthcare becomes more efficient, equitable, and patient-focused in the years to come. As AI technologies continue to evolve, they hold the promise of addressing global healthcare challenges and improving accessibility for underserved populations.
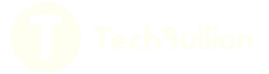