At TechBullion, we’re thrilled to bring you an exclusive conversation with Raghu Para, a distinguished AI/ML specialist and a Full Stack Architect with over 15 years of groundbreaking contributions to technology and artificial intelligence. Known for his expertise in engineering, analytics, and natural language processing, Para has helped companies across Asia, Australia, and North America redefine innovation and achieve transformative results. With a multidisciplinary background spanning technology, journalism, and digital media ventures, Para offers a unique lens on the intersection of AI and everyday life. In this interview, he delves into the fascinating world of discrete intelligence, exploring how AI systems can work seamlessly in the background to enhance human experiences while maintaining ethical and personalized outcomes.
Luke: Raghu Para, thank you for joining us today. Your work in AI/ML and technical product management is widely recognized. Let’s start with the basics: what is invisible intelligence, and how is it shaping the way we interact with technology?
Para: Thank you for having me! Invisible intelligence refers to the seamless integration of AI into our daily environments, where the technology operates quietly in the background, intuitively understanding and anticipating human needs. It’s about AI systems becoming less intrusive and more intuitive, creating experiences that feel natural rather than engineered.
Take the concept of ambient intelligence as an example. This involves interconnected systems—smart sensors, devices, and AI models—that adapt to users without requiring explicit commands. You see it in smart homes, where the lights adjust to your mood, or in smart cities, where traffic is dynamically rerouted to reduce congestion. Invisible intelligence allows AI to function as a silent collaborator, enhancing human convenience while fading into the background.
Luke: That sounds futuristic but also very real. Can you share specific examples of how invisible intelligence is already being applied in smart cities and smart homes?
Para: Absolutely. In smart cities, you’ll find invisible intelligence driving systems like adaptive traffic management. AI analyzes live traffic data, predicts congestion, and adjusts signals dynamically to optimize flow. Public transit systems leverage similar technology to predict delays and reroute buses in real time, ensuring smoother commutes.
In smart homes, the best examples come from integrated systems like voice assistants and IoT devices. But beyond the voice interface, many AI-driven features are entirely silent. For instance, a thermostat that learns your temperature preferences over time and adjusts without you noticing, or refrigerators that track food expiration dates and suggest recipes based on available ingredients. These systems demonstrate how invisible intelligence can blend into daily life without requiring constant interaction.
Luke: Fascinating. From a technical standpoint, what makes this discreet integration of AI possible? What’s happening under the hood?
Para: Great, and an important question. At its core, invisible intelligence relies on advancements in several key areas:
Edge Computing: By processing data locally, close to where it’s generated, devices can make quick decisions without needing constant connectivity to the cloud. This reduces latency and enhances privacy.
Sensor Fusion: Combining inputs from multiple sensors—like cameras, motion detectors, and microphones—creates a comprehensive understanding of the environment. For example, a smart home system might combine motion data with ambient light levels to decide when to dim the lights.
Machine Learning Models: These include large language models (LLMs), computer vision algorithms, and reinforcement learning agents that continuously learn and adapt to user behaviors.
Context Awareness: AI systems now have a better grasp of context, thanks to advancements in natural language processing (NLP) and computational linguistics. This means systems can interpret user needs based on location, time, and prior behaviors.
Cloud and Big Data Integration: Invisible intelligence wouldn’t be possible without vast amounts of data to train and fine-tune these models. Cloud computing provides the storage and computational power to analyze and derive insights from this data.
Luke: How do you see invisible intelligence evolving over the next five years?
Para: The next five years will see invisible intelligence becoming even more pervasive and refined. Here are some key trends I’ve been working on, and I think will be critical in shaping the future:
Hyper-Personalization: AI systems will better understand individual preferences and adapt accordingly. For instance, in smart homes, devices will learn your routines down to micro-levels, like knowing when you prefer coffee versus tea.
Seamless Interoperability: As more devices and platforms adopt standardized protocols, AI systems will communicate more effectively. This means your car, home, and workplace will sync effortlessly to provide a unified experience.
Proactive Assistance: Instead of reacting to commands, AI will anticipate needs. For example, a smart city system might reroute you before you hit traffic, or a home assistant might schedule maintenance when it detects appliance issues.
Ethical and Transparent AI: With increasing adoption comes a greater focus on privacy and ethics. Invisible intelligence systems will need to be transparent about data collection and decision-making processes to maintain user trust.
Applications in Healthcare: Invisible intelligence will revolutionize preventive care. Wearable devices will quietly monitor vitals and detect anomalies, alerting users or healthcare providers only when necessary.
Luke: Speaking of privacy, the idea of AI working silently in the background raises concerns about data usage. How do we ensure that invisible intelligence respects user privacy?
Para: Privacy is indeed one of the biggest challenges. Here’s how we can address it:
Data Minimization: AI systems should only collect and process data that is absolutely necessary for their function.
Federated Learning: This technique allows AI models to learn from data distributed across devices without transferring it to a central server. It’s widely used in applications like keyboard suggestions and helps maintain user privacy.
Edge Intelligence: By processing data locally on edge devices, we can reduce the need to transmit sensitive information to the cloud.
Encryption and Secure Protocols: All data, whether in transit or at rest, must be encrypted. Additionally, organizations should adopt secure protocols for device communication.
User Control: Transparency and control are critical. Users must be informed about what data is being collected and given the ability to opt out or delete their data.
Privacy isn’t just about compliance—it’s about trust. For invisible intelligence to thrive, users need to feel confident that their data is safe and being used responsibly.
Luke: You’ve mentioned large language models (LLMs) like GPT or similar technologies. How do they fit into the concept of invisible intelligence?
Para: LLMs are a cornerstone of invisible intelligence, especially in systems that require understanding and responding to human language. For instance:
In Smart Homes: LLMs power conversational interfaces, enabling devices to understand natural language commands or even interpret implicit requests, like “It’s cold in here,” and adjust the temperature.
In Smart Cities: LLMs analyze large volumes of textual data, such as social media posts, to predict trends or identify areas needing attention during emergencies.
Proactive Assistance: By analyzing historical data, LLMs can predict user needs and offer suggestions without being prompted.
The beauty of LLMs lies in their versatility—they can process unstructured data, learn user preferences over time, and enhance the contextual awareness of AI systems.
Luke: Let’s shift gears to the role of businesses. How can companies leverage invisible intelligence to improve customer experience and operational efficiency?
Para: Invisible intelligence offers tremendous potential for businesses. Here’s how it can be applied:
Customer Experience: Retailers can use ambient AI to personalize in-store experiences. Imagine walking into a store, and the digital signage adapts to your preferences based on prior purchases or loyalty app interactions.
Operational Efficiency: In logistics, ambient intelligence can optimize warehouse operations. AI-powered sensors can track inventory levels and automate restocking, reducing manual effort.
Energy Management: Businesses operating smart offices can use invisible intelligence to optimize energy usage by adjusting lighting, heating, or cooling based on occupancy and schedules.
Predictive Maintenance: AI can monitor equipment health in real-time and schedule maintenance proactively, preventing costly downtimes.
Employee Productivity: In the workplace, AI assistants can help employees prioritize tasks, schedule meetings efficiently, and even suggest relevant information during discussions.
The key for businesses is to align AI capabilities with real-world problems and deploy them in ways that feel natural and intuitive.
Luke: What are some of the biggest challenges you foresee in scaling invisible intelligence across industries?
Para: Scaling invisible intelligence isn’t without its hurdles. Some of the biggest challenges include:
Interoperability: Many devices and platforms still operate in silos. Standardizing communication protocols will be essential for seamless integration.
Cost and Accessibility: Advanced AI systems can be expensive to develop and deploy. Democratizing access to these technologies will be critical for widespread adoption.
Ethical Concerns: The more invisible AI becomes, the greater the risk of it being used for manipulative or unethical purposes. Robust governance frameworks will be needed to ensure fair use.
Technical Complexity: Designing AI systems that are context-aware, adaptive, and lightweight enough to operate on edge devices requires overcoming significant engineering challenges.
Consumer Trust: Adoption will depend heavily on public perception. Educating users about the benefits and safeguards of invisible intelligence will be key.
Luke: Finally, if you were to give one piece of advice to AI professionals aspiring to innovate in this space, what would it be?
Para: My advice would be to focus on human-centric design. Technology should enhance human experiences, not complicate them. To achieve this:
Always start with the user’s perspective. Understand their needs, behaviors, and pain points.
Keep solutions simple and intuitive. Invisible intelligence works best when it feels effortless.
Stay committed to ethical principles. Innovation should never come at the expense of privacy or fairness.
Above all, remember that invisible intelligence is about creating a harmonious relationship between humans and technology—one where AI enhances our lives without demanding our constant attention.
Luke: Thank you, Mr. X, for this insightful conversation. Your thoughts on invisible intelligence are both inspiring and thought-provoking!
Para: Thank you! It’s been a pleasure sharing my views on this exciting frontier in AI. I’m looking forward to seeing how this field evolves in the coming years.
This interview captures the technical depth, visionary insights, and real-world applications of invisible intelligence while keeping the tone accessible and engaging for a broad audience.
Connect with Raghu Para on LinkedIn here.
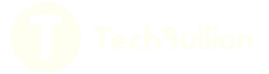