In the world of heavy machinery, minimizing downtime and maximizing fleet performance are not just operational goals, they are critical to a company’s bottom line and customer satisfaction. Using data science and predictive analytics can help companies to forecast component failures, reduce maintenance costs, and improve fleet reliability. This would ensure better service and customer loyalty.
Such a strategic approach is emphasized by an industry expert, Srujana Manigonda, whose experience from a project focused on life-limiting components in engines and drivetrain systems has provided unique insights into how data science can play a key role in extending the life of these components and improving overall fleet performance.
Manigonda has also shared her insights in the article, “Empowering Data-Driven Decision Making in Manufacturing,” where she discussesthat how in manufacturing, data-driven decision-making (DDDM) enhances productivity, reduces costs, and improves quality. This approach, focused on predictive maintenance, supply chain optimization, and quality control, addresses challenges like data silos and workforce adaptability, enabling manufacturers to thrive in Industry 4.0.
Understanding the Challenge
As highlighted by the professional, heavy equipment like engines and drivetrains are often exposed to extreme conditions that accelerate wear and tear on certain components. Over time, certain parts of the machinery experience failure due to age, usage, and environmental factors. These failures can lead to significant operational downtime, high repair costs, and most importantly, frustrated customers.
The key challenge in addressing these issues is identifying which components are likely to fail and when. This allows for preventive measures, such as predictive maintenance, to extend their lifespan and reduce unexpected breakdowns.
During her time working on this project, she was responsible for contributing independently as part of a multi-person analytics team. Her team focused on life-limiting components within the machinery, and the goal was to develop a consistent and proven process to extend their life cycles. This work brought immediate results, saving millions in downtime costs and drastically improving maintenance efficiency across the fleet.
How Data Science Played a Crucial Role
Manigonda realized that historical data could be extremely valuable in predicting future outcomes. She had access to a wealth of operational data including engine usage statistics, maintenance logs, environmental conditions, and component performance over time. She worked on analyzing this data and developing predictive models to forecast the failure of life-limiting components.
Using machine learning algorithms, she assisting in creating models that identified patterns in the data, enabling the organization to predict when a component was likely to fail. By analyzing historical trends, the best time to perform maintenance could be determined, minimizing the chances of unplanned failures. The predictive model also empowered the maintenance team to order parts in advance, schedule repairs more effectively, and allocate resources more efficiently, ultimately keeping machines running longer and reducing unnecessary downtime.
These contributions have driven impactful results for her firm. Mentioning the impacts, the professional stated, “I contributed to the development of predictive models that accurately forecasted the life expectancy of critical components, helping reduce unexpected equipment failures and minimize downtime.” The insights gained from the predictive models led to significant cost savings. Since maintenance activities were scheduled proactively, millions in repair costs were saved and unplanned downtime was significantly reduced.
By extending the life of critical components and ensuring they were maintained before failure, fleet performance was also enhanced. Machinery experienced fewer breakdowns, operated at optimal capacity for longer periods, and provided more consistent service to customers.Predictive models significantly reduced emergency repairs, lowered costs, and improved resource utilization efficiency, driving greater operational effectiveness.The impact on customer satisfaction was another output. By preventing unplanned failures and ensuring the fleet operated smoothly, the team delivered more reliable machines to customers, which strengthened customer loyalty and enhanced brand reputation.
Future Trends and Insights
As IoT devices become more sophisticated, more real-time data will be available, allowing for more precise predictions. The integration of AI and ML in maintenance practices will become increasingly common, enabling even smarter systems that learn from new data as it becomes available.
Moreover, the future of fleet management will likely see the development of fully autonomous systems that not only predict maintenance needs but also automatically adjust operational parameters to prolong component life. This will be especially beneficial in reducing human error and ensuring that machinery operates at peak efficiency with minimal intervention.
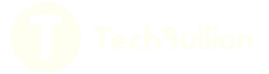