The automotive market is one of the leading sectors driving AI growth and innovation. In 2025, almost 60 percent of all new cars sold globally will have Level 2 autonomy. In 2030, Level 2 autonomous vehicles are still expected to dominate the market, but Level 3 and Level 4 autonomous vehicles should account for around eight percent of all new car sales. The demand for autonomous driving technologies, predictive maintenance, and connected car features fuels this growth.
Why does the automotive industry hold such potential for AI advancements? How will data labeling approaches evolve to meet this rise? Why do the latest smart solutions and models raise concerns about quality, calling for improvements? And why are foundational methods once again becoming essential for creating high-quality datasets?
The Keymakr CEO Michael Abramov sheds light on these pressing questions.
Reasons Behind Rapid Growth
The automotive industry is poised for continued active development, and the explanation is entirely logical. This sector already has a well-established infrastructure: roads, vehicles, and materials like asphalt and rubber that have been produced for a long time. This provides a solid foundation for integrating new technologies. For instance, improving the existing system can often be achieved by adding software, cameras, or sensors.
Compare this with robotics, where the situation is much more complex. There are no robots yet that can move freely and efficiently through spaces, nor is there an infrastructure for charging them or safely integrating them into public areas. Even basic elements, like dedicated pathways or zones to avoid conflicts with pedestrians, are absent. This industry requires significantly more time and investment to develop.
A similar challenge exists with drones. Currently, there is almost no civilian infrastructure to support their widespread use. Operating drones involves numerous restrictions, such as the need for licenses, insurance policies, and, critically, the lack of clear regulations and accountability frameworks.
In contrast, the automotive industry is already primed for further innovation. It has an existing foundation upon which new technologies can be layered, making it an obvious leader for near-term growth.
Adapting to Change: How Data Labeling Addresses the Dynamic Automotive Ecosystem
However, having a solid background in the automotive industry doesn’t guarantee 100% success in creating rapid and innovative solutions. Continuous and meticulous image and video annotation is essential, primarily due to the need to meet high precision standards and adapt to the ever-changing environment.
A high level of precision is crucial for ADAS (Advanced Driver Assistance Systems) models because errors can have catastrophic consequences. For instance, if an autonomous vehicle system misidentifies a pedestrian or fails to avoid hitting them in favor of striking a pole, it could result in a global tragedy. This is why the automotive sector focuses on the “Critical Path,” where achieving increasingly high precision levels (e.g., 99.9%, 99.9999%) becomes a top priority.
Achieving such results, however, is becoming more challenging due to the constant evolution of the environment. Several factors influence this evolution:
- Vehicle Design: Manufacturers update their models’ designs every three years through facelifts. For example, the Mazda 3 has undergone multiple design changes. These updates require continually retraining machine learning models to recognize new shapes accurately.
- Infrastructure: Roads, markings, and traffic signals also change. For instance, road markings are now made matte to reduce slipperiness, but reflective materials might become standard in the future. Such updates demand algorithm adjustments.
- Urban Environment: Even small changes, such as replacing one type of tree with another (e.g., poplars with maples), can affect model performance. Variations in lighting, building architecture, road surfaces, or traffic lights necessitate adaptations.
- Human Factors: People’s appearance, fashion trends, and behaviors are also part of this evolving environment. For example, if purple hair becomes a popular trend, recognition systems might struggle to distinguish pedestrians from other objects.
This constant evolution makes data labeling both necessary and perpetual. New data is needed to train models to account for changes in the surrounding world. Additionally, advancements in materials, technologies, and algorithms require system updates to improve accuracy and performance.
Ultimately, the data labeling approach will continue evolving to meet growing demands, ensuring safety and precision across diverse conditions.
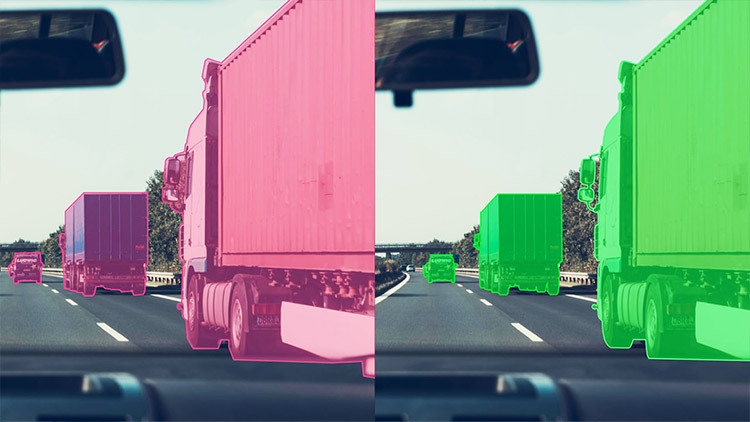
Credit: Keymakr
Will Automation Replace Manual Data Annotation?
The decline in demand for manual data annotation is undeniable, and this trend is evident. In the past, the data labeling industry saw rapid growth, with thousands or even tens of thousands of annotators in demand. Today, however, a significant portion of tasks have been automated.
For instance, facial recognition technology almost no longer requires image annotation because the problem is essentially solved, and faces don’t evolve as dynamically as, say, vehicles.
The mass market has shifted toward automation and the use of universal models like YOLO and SAM. Recently, the release of Samurai has, in some ways, revolutionized the process. These models simplify routine and repetitive tasks.
In the past, companies like Tesla invested massive budgets in data annotation to train their models. Now, they can use open-source tools to handle tasks that once required significant resources.
However, there’s an important caveat: these models are limited by their generalization. Such systems are suitable for automating standard and uniform operations, but complex or unique cases still require human attention. Human involvement in data labeling remains the cornerstone of accuracy and quality. Sometimes, there’s no substitute for someone picking up a mouse and manually annotating data.
The data annotation industry, in some way,s resembles the watch market. Mechanical watches, like Rolex, lost their practical necessity long ago. Smart devices have taken their place, offering not only timekeeping but also features like heart rate monitoring, step counting, and temperature tracking. Yet, despite the widespread adoption of “smart” technologies, the mechanical watch segment remains in demand.
Why? It’s the boutique effect. Scientists value uniqueness and quality.
For this reason, there will always be a place for boutique solutions tailored to unique tasks requiring individual approaches. Companies specializing in high-precision images and video annotation of complex and non-standard data will remain relevant. Thus, the key trend is shifting from mass data labeling to providing niche services, where quality, precision, and attention to detail take center stage.
From Cost-Cutting to Quality Concerns: The Shift Back to Rigorous Data Annotation
Over the past three years, as the image annotation industry shifted heavily toward automation, the volume of manually annotated data used to train models decreased. However, this approach has led to regression. The quality of model performance didn’t improve as much as expected, becoming apparent to clients.
The automotive market has unique characteristics. Deals with OEM manufacturers (Volkswagen, Ford, Mercedes, Porsche) are typically long-term, with cycles often spanning 1.5 – 2 years. OEMs — vehicle manufacturers — are extremely meticulous in selecting AI solutions due to strict regulations, certifications, and standardization requirements. For example, contract negotiations might begin in 2022, the contract is signed in 2024, and integration doesn’t start until 2025. However, solutions based on 2023 technologies are often obsolete by the time they are implemented.
This challenge has prompted companies developing ADAS (Advanced Driver Assistance Systems) solutions to realize that their models, sold a few years ago, are no longer performing adequately. To address this, they must urgently return to more comprehensive data labeling and retraining processes.
The entire autonomous vehicle industry now faces a similar challenge. Quickly addressing quality issues is not feasible. The process requires significant resources and time. Companies that once believed minimal effort was sufficient to maintain model performance now recognize how rapidly technologies and requirements evolve. They are being forced to allocate far more resources to remain competitive and deliver high-quality solutions.
The AV industry is undergoing a period of reassessment, returning to foundational methods and placing renewed emphasis on high-quality data labeling.
As data annotation providers, it’s essential for Keymakr to remain flexible and continually seek new solutions to stay relevant in this rapidly evolving technological landscape. The importance of high-quality data cannot be overstated, and our adaptability will be key to meeting the demands of the future.
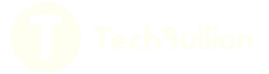