Financial expert Neeraj Kripalani, based in the USA, has published research on how artificial intelligence is transforming financial strategy through continuous monitoring systems. His work reveals significant advancements in AI-driven financial analysis and decision-making processes.
The Future of Financial Decision-Making
Recent innovations in AI-driven financial monitoring are revolutionizing how organizations approach cost management and profitability enhancement. Traditional methods often struggle with processing vast amounts of data efficiently, leading to missed opportunities for cost optimization. Organizations implementing AI-driven financial monitoring systems have reported an average 15-20% reduction in operational costs within their first year of deployment, marking a significant shift in financial management capabilities.
Smart Systems, Smarter Decisions
The integration of sophisticated pattern recognition algorithms and deep neural networks has transformed financial analysis capabilities. These systems excel at identifying recurring spending patterns, seasonal variations, and anomalous transactions while processing both structured and unstructured financial data. The implementation of time series analysis using Long Short-Term Memory networks enables the capture of complex temporal dependencies in financial data, providing unprecedented insights into spending trends and future financial patterns.
Precision Through Automation
Modern AI systems have achieved remarkable accuracy in financial operations, with supervised learning models reaching up to 95% accuracy in expense categorization and anomaly detection. This represents a significant improvement over traditional rule-based systems, enabling organizations to make more informed decisions about resource allocation and budget optimization. The enhanced precision in financial monitoring has led to more effective risk management and improved compliance tracking.
Real-Time Monitoring Revolutionizes Resource Management
The research reveals several critical findings in resource utilization, including a 23% underutilization of licensed software applications and various opportunities for shared resource pooling. AI systems can now identify peak usage periods requiring optimization and detect dormant assets and services, leading to more efficient resource allocation. This real-time monitoring capability enables organizations to respond swiftly to changing financial conditions and market dynamics.
Budget Optimization Gets Smarter
AI-driven budget optimization has revolutionized financial management, yielding an 18% average improvement in budget efficiency across organizations. These sophisticated systems analyze historical data and future projections to recommend strategic budget reallocations. By employing dynamic allocation models and tracking spending patterns, the AI provides actionable insights for financial decision-makers. The technology’s machine learning capabilities ensure continuous improvement, as algorithms refine their predictive accuracy through ongoing data analysis, leading to increasingly precise budget optimization recommendations.
Implementation: A Structured Approach
The implementation of AI-driven financial monitoring demands a methodical, phased approach for optimal results. Organizations that adopt a gradual deployment strategy achieve 40% higher success rates in AI integration compared to those pursuing aggressive full-scale implementation. The recommended 8-12 month rollout begins with foundational setup, progresses through pattern recognition development, and culminates in sophisticated analytics and optimization capabilities. This measured timeline allows teams to adapt naturally, maintain operational continuity, and systematically validate each phase before advancing, ultimately ensuring a more robust and sustainable integration of AI monitoring systems.
Measuring Success
AI implementation has revolutionized financial performance metrics across organizations. Software license utilization has seen a dramatic increase from 77% to 95%, while spending categorization accuracy has jumped from 75% to 93%. These significant improvements highlight AI’s transformative impact on financial monitoring. The results extend beyond raw numbers, with organizations experiencing enhanced decision-making capabilities, substantial cost reductions, and more efficient resource distribution throughout their departments.
Future Horizons
The evolution of financial monitoring systems continues with emerging opportunities in advanced algorithm development, blockchain technology integration, and cross-organizational data sharing frameworks. These developments promise even greater capabilities in predictive analytics, risk assessment, and automated decision-making. The integration of AI with other emerging technologies is expected to create more sophisticated and comprehensive financial monitoring solutions.
In conclusion, the integration of AI in financial monitoring represents a pivotal shift in organizational management practices. The comprehensive research by Neeraj Kripalani highlights that despite implementation challenges, AI-driven systems deliver substantial benefits through enhanced predictive capabilities and automated decision-making. As financial landscapes become increasingly complex, these systems prove essential for maintaining competitive advantage. The future promises even more sophisticated AI applications, transforming financial monitoring into a strategic asset that delivers improved efficiency, accuracy, and long-term financial health for organizations worldwide.
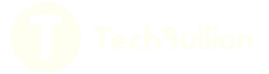