Background and Introduction
As P&C insurance Underwriting and Claims environment becomes saturated with data, conventional risk assessment methods are no longer sufficient in supporting accurate decision-making. Big data analytics has created a new horizon where different forms of data including social media, sensor data, geographical data, and environmental data, are included in risk models. This study examines a number of big data analytics approaches, methods, and strategies—including Machine Learning, Predictive Modelling, and Artificial Intelligence—in relation to P&C insurance.
Risk management process in Property & Casualty (P&C) insurance facilitates an insurer to assess, value and control expense probable from an event. It is crucial in selecting risks and underwriting since it allows insurers define insurable hazards and keep portfolio earnings up by matching prospective risk with the Underwriting Appetite. In addition to underwriting and rating, risk assessment helps insurers manage exposures and recommends ways of reducing future claims’ occurrence and cost, which would provide value for both insurers and policyholders through loss control.
Traditionally, risk assessment in P&C insurers relied heavily on the regional or national industrial data, historical claims experience, actuarial calculations, and above all underwriting judgement. However, with big data analytics, insurers are able to construct a new system of analytics by collecting huge amounts of data from a variety of related and unrelated sources, such as IoT (Internet of Things) devices and gadgets, Social Media, Weather reports and even consumer behavior. For instance, usage based insurance pricing achieved through telematics in auto insurance by obtaining driving information in real-time helps insurers set appropriate premiums pegged on customer driving habits rather than demographics. Customers are also benefitting because more customer-oriented insurance products have been created targeted to their unique needs.
“Big Data” is a relatively new phrase for datasets that goes beyond the capabilities of conventional database management systems. These data sets are so large that standard software and storage solutions cannot adequately collect, store, manage, or analyze them in a reasonable amount of time [1]. Companies may now use big data analytics to sift through a variety of data types, including structured, semi-structured, and unstructured information, in pursuit of actionable insights [2].
Utilizing Big Data Analytics in P&C Insurance Risk Management
Deploying advanced analytics on large data sets is critical in improving risk evaluation in the Property and Casualty (P&C) insurance industry. Through use of both structured and unstructured data, insurance organizations can achieve broader depths of risk and market understanding. Here’s a detailed overview of how big data analytics is applied in risk assessment within the P&C insurance sector:
Improved Risk Modeling
- Predictive Analytics: Predictive models are employed in the insurance industry to make insurer predictions regarding past data that is most likely to exhibit a certain risk. Therefore, using some form of Machine Learning algorithms, insurers can forecast future claims and create estimate models and patterns of possible financial losses.
- Advanced Statistical Techniques: Advanced analytics tools like regression analysis, clustering and classification functions to put various risk indicators and categorize customers for the insurers, are getting popularity with today’s insurers.
Enhanced Underwriting Processes
- Data Integration: Big data analytics also enable the inclusion of external data, in addition to internally produced telematics data and IoT-generated data, which leads to improved risk assessment.
- Dynamic Pricing Models: Insurers can use real-time data to design time varying tariffs that can reflect an individual risk profile rather than just an average risk.
Real-Time Risk Assessment
- Telematics and IoT Data: Telematics and IoT devices collect behavior and conditions of vehicles as well as homes in real time through constant supervision. Dynamic risk assessment can be done, meaning insurers can intervene timely and change policy terms as necessary.
- Event-Driven Analytics: Closely linked to this, insurers can gain real-time feed from weather services or social media to review the risks pertaining to natural catastrophes or new threats and improve their sensitivity.
Fraud Detection and Prevention
- Anomaly Detection: Sophisticated means of analyzing claim data can reveal abnormality that is associated with fraud. This helps several companies where the machine learning algorithm is applied can himself claims for the further investigation of past fraud patterns.
- Social Network Analysis: The general exploitation of relations between the participants and other individuals involved can reveal fraudulent schemes and increase the effectiveness of risk assessment.
Enhanced Customer Insights
- Behavioral Analytics: Taking into consideration certain aspects of customer behavior, insurers can predict those individuals who are most likely to claim and design the products to meet these particular profiles.
- Sentiment Analysis: Engaging with the public through the social media platforms and analyzing feedback rendered by customer also assist insurers in understanding sentiments of the public that may impact risks inherent in their products.
Geographic Risk Assessment
- Geospatial Analytics: Spatial technologies such as GIS support risk visualization and give an insight into risks associated with specific regions, for example, flood-prone areas or areas at high risk of natural disasters.
- Climate Data: While evaluating climate characteristics and studying historical weather conditions, insurers find it easier to estimate the connected risk and thus price services more effectively.
Claims Analytics
- Claims Predictability: Backward claims data may be employed to forecast the next possible claims that might be made so that the insurers can prepare adequately to handle claims that might be made in future.
- Loss Severity Analysis: Justifying claims related to past losses to understand trends in loss severity is efficient in handling probable changes to assessments and reserves.
Paper Review
Here are some related works showcasing how big data analytics can be used to enhance risk evaluation process.
1) In, Londhe and Prasada Rao, (2018) a review of the different Big Data analytics software frameworks is conducted, and a thorough evaluation of their advantages and disadvantages is examined. Furthermore, popular data mining algorithms are examined for their applicability for addressing real-world application issues in relation to their adaption for Big Data analysis. By taking into account the strengths of existing software frameworks and platforms, we can conduct in-depth analyses of different platforms that are well-suited for Big Data processing, and thus forecast future trends in Big Data analytics and processing[3].
2) In, Subbalakshmi and Prabhu, (2018) discusses writing about Big Data and Predictive Analytics in great detail. In this emerging subject, it provides unadorned elements of pressing thinking. Experts and academics now consider big data to be a crucial area of interest for study and research. Large-scale data storage and analysis is now cheaply possible because of technological advancements. Starting from a variety of sources, Enormous Data compiles a mixture of written, partially organized, and unstructured progressive data. In a similar vein, big data creates a few issues, particularly with regard to estimation, quality, consistency, and data satisfaction[4].
3) In, Lee, (2013) examines the results of company questionnaires and interviews to support the model employing structural equation modelling, evaluates past customer questionnaire outcomes for fire insurance and fire security protection, and uses an integrated approach to draw conclusions about the factors influencing strategic alliances. This paper proposes a model of strategic alliances for heterogeneous property and casualty insurance and security companies, along with a value chain and contracting strategies linked to value chain analysis. It also adds a seventh business function called “business expansion” to address consumer demand for integrated services[5].
Conclusion
The integration of Big Data Analytics (BDA) into the property and casualty (P&C) insurance sector has transformed risk assessment, enabling insurers to analyze vast volumes of structured and unstructured data for informed and precise, data-driven decision-making. Big data analytics help insurers to model risk more accurately, identify frauds, and develop new products. In particular, Machine Learning and Predictive Analytics are instrumental in trend detection and risk assessment, while the unstructured data analytics gives a broader view of consumers and the environment. Although, challenges such as the investment in structures, security of data, and legal issues have to be addressed for adoption to work, today big data analytics is critical to P&C insurance industry’s ongoing evolution and offers potential ways to increase performance and minimize threats and opportunities to improve customer satisfaction.
References
[1] W. R. Kubick, “Big Data, Information and Meaning,” Appl. Clin. Trials, 2012.
[2] P. Gautam and Y. P. S. gh, “Impact of Data Mining on Big Data Analytics: Challenges and Opportunities,” Int. J. Comput. Trends Technol., 2018, doi: 10.14445/22312803/ijctt-v57p104.
[3] A. Londhe and P. V. R. D. Prasada Rao, “Platforms for big data analytics: Trend towards hybrid era,” in 2017 International Conference on Energy, Communication, Data Analytics and Soft Computing, ICECDS 2017, 2018. doi: 10.1109/ICECDS.2017.8390056.
[4] S. Subbalakshmi and C. S. R. Prabhu, “Protagonist of Big Data and Predictive Analytics using data analytics,” in Proceedings of the International Conference on Computational Techniques, Electronics and Mechanical Systems, CTEMS 2018, 2018. doi: 10.1109/CTEMS.2018.8769141.
[5] J. C. Lee, “An international value chain via strategic alliances with an integrated approach: Property & casualty insurance companies and security companies,” in 2013 10th International Conference on Service Systems and Service Management – Proceedings of ICSSSM 2013, 2013. doi: 10.1109/ICSSSM.2013.6602503.
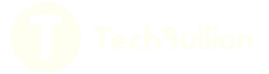