Predictive analytics has emerged as a game-changing solution in modern business. It uses data to make predictions about future outcomes. It enables a company to predict customer trends, behaviors, and preferences by analyzing the historical data for making better decisions. Indeed, delivering a personalized customer experience is something very crucial for every business in today’s competitive marketplace to enhance engagement and loyalty. In fact, today’s customers expect their interactions to be unique and personalized. Personalization is now a vital differentiator. Predictive analytics helps organizations match this expectation with applicative abilities such as segmentation of customers, behavior analysis, and retention strategies.
What is Predictive Analytics in Customer Experience?
Predictive analytics involves the use of historical data, statistical algorithms, and machine learning techniques in predicting future customer behaviors and outcomes. This way, a business may anticipate what customers are likely to do next when patterns from past customer interactions are realized, including likely purchases a customer is going to make, preferences, and overall engagement.
Predictive analytics solutions are inseparable from AI and ML. These technologies make it automatic to analyze data, hence increasing the accuracy of the prediction, and also enable business scaling by personalizing their offerings. AI algorithms learn with new data and keep refining their insights over a period.
Real-world examples show that predictive analytics improve customer experiences. Amazon uses the system to suggest products based on one’s surfing history and purchasing habits. Netflix uses predictive models to offer movies or series that best fit the viewing habit of users, while Spotify creates playlists based on a person’s preference in music.
Tailoring Products and Services with Predictive Analytics
Customer Segmentation
The other use of predictive analytics could be customer segmentation, thus helping businesses with targeted marketing campaigns based on behavior, demographics, and preference. Clustering algorithms may help companies in refining their customer segmentation further by extracting micro-groups within their customer base for hyper-personalized product recommendations, thereby crafting a more relevant user experience.
For instance, a retail company can use predictive analytics to study purchase history and browsing for customer grouping. Since it groups customers who buy similar products routinely, it can send recommendations that each particular group relates to, hence converting them.
Behavioral Analysis
Predictive analytics can analyze browsing patterns and buying behavior to predict what a customer may want before a conscious decision is made. Processing data in real time for AI-driven insight means business can offer products or services at the most opportune moments. As in e-commerce websites, Amazon receives several intent signals through search terms or time spent over a product page and recommends similar products to raise the chances of a sale.
Predictive Analytics in Marketing Strategies
Predictive analytics is making marketing strategies completely different; it helps companies to run very targeted campaigns. A company can determine the best channel, timing, and message for an individual customer based on the analysis of past interactions and behavior. It might define, for instance, the moments when an individual customer is likely to open emails and thus help in conducting email marketing campaigns more effectively.
This also optimizes ad targeting so that customers only see the ads that interest them and fit their needs. Predictive analytics also contributes to personalization in website and app content by allowing customers to see products and services only relevant to their preferences and behaviors.
Cross-Selling & Upselling
Predictive analytics leads to important insights into cross-selling and upselling. It analyses purchase patterns where businesses can recommend complementary or upgraded products to customers. Predictive models identify when customers are most likely ready for additional purchases, allowing them to deliver targeted offers at just the right moment. This could be something as simple as how predictive analytics at Amazon determines the “frequently bought together” recommendations when users add more items to their cart.
Customer Retention Strategies with Predictive Analytics
Customer churn is a challenge that most businesses have to confront. Predictive analytics identifies such at-risk customers by analyzing the trend in the customer’s behaviors that show reduced engagement, slower response times, or decreased purchasing activity. Once such patterns are identified, businesses can take early steps to intervene and prevent churn with loyalty rewards, special discounts, or personalized offers.
One perfect example of how predictive models are put to work to avoid churn is in telecom companies. Through tracking usage habits and calling or visiting habits when customers call or visit their customer service, the telecom providers can try to pick up signals of dissatisfaction and proactively sort out the problem to retain their customer base.
Loyalty and Lifetime Value
Predictive analytics enables the firms also to predict CLV, which thereby helps them in concentrating their efforts on high-value customers. This allows the businesses to understand which customers are most likely to deliver value over time, so they can focus on personalized rewards or exclusive offers as part of building a relationship with them.
For example, a subscription service might use predictive analytics to identify which customers are most likely to upgrade to premium plans. The business can then easily offer highly valued incentives to such customers through increased loyalty.
Implementing Predictive Analytics in Your Business
Here’s how you can integrate predictive analytics into your business model:
- Data Collection: Aggregate data from all valid customer touch-points, which include sales, social media interactions, website traffic, and customer service records.
- Model Development: The development of statistical models to analyze the patterns, using techniques in machine learning.
- Solution Tools & Platforms: SAS, Google Analytics, Salesforce Einstein, amongst others, to harness the best outcome for the need of business.
It is also very important that the organization instills a data-driven culture, coupled with skilled teams that know exactly how to manage and interpret predictive models. Only a clear focus on continuous learning and optimization will ensure successful implementation to drive results.
To Sum Up
In all, predictive analytics is an indispensable tool for any business willing to provide personalized customer experiences. By making proper adjustments to product and service offerings, along with methods of marketing, companies can considerably enhance consumer engagement and loyalty. Be it through segmentation, behavioral analysis, or retention strategies, predictive analytics lets business houses engage with customers by anticipating their needs and building long-term relationships. Companies have no other choice but to implement predictive analytics and use it to the fullest if they want to stay competitive and meet customers’ evolving expectations.
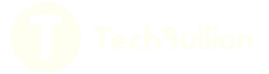