Renewable energy and sustainable resource management play crucial roles in the face of climate change. Creating well-optimised processes for efficient energy management is a complex task. However, statistics show that advanced technologies such as artificial intelligence (AI) and machine learning (ML) are increasingly significant in optimising and improving green energy management. This article will discuss essential opportunities and challenges these technologies can offer the sustainable energy sector.
What Are the Critical Opportunities for Artificial Intelligence and Machine Learning in Renewable Energy Sector?
AI algorithms and ML models can automate various tasks, including collecting and analysing data. It creates many possibilities for improving the performance and management of renewable energy sources. Here are the most essential of them.
1. Energy Production Optimisation
Integrating artificial intelligence and machine learning into renewable energy can create an excellent opportunity to optimise energy production. These technologies can analyse vast amounts of data from various sources, such as weather forecasts, historical performance data, and real-time sensor inputs, to enhance the efficiency and effectiveness of renewable energy systems. There are several ways to achieve it, including:
Weather Forecasting
Machine learning algorithms, such as neural networks and regression models, can accurately predict weather conditions. These predictions help determine the optimal times for production. Accurate forecasts improve production management by optimising solar panel positioning for maximum sunlight capture and allowing turbines to adjust blade angles for optimal wind capture.
Demand Prediction
Time series analysis, clustering algorithms, and deep learning models can analyse past energy consumption patterns to forecast future demand. Forecasting energy demand ensures that renewable energy sources can meet consumption needs without overproducing or underproducing. This balance helps reduce waste and improve the sustainability of energy resources.
Grid Condition Analysis
Real-time data analysis, reinforcement learning, and optimisation algorithms can monitor and manage grid conditions. By understanding these conditions, AI systems can adjust production rates to maintain grid stability and prevent outages. This dynamic adjustment helps integrate variable renewable energy sources like wind and solar into the grid.
2. Predictive Maintenance
AI shows great potential for enhancing maintenance processes in renewable energy by analysing data from various interconnected sensors installed on equipment such as wind turbines and solar panels. With the help of ML algorithms, AI can predict when maintenance is needed, preventing equipment failures, reducing downtime and extending lifespan.
The technique involves anomaly detection models that can process data from sensors that monitor equipment conditions like vibrations, temperature, and performance metrics. Early detection of anomalies allows for timely maintenance, preventing minor issues from escalating into significant failures and keeping optimal equipment performance. It minimises downtime, reduces maintenance costs, and extends the operational life of renewable energy assets.
For example, AI can detect unusual vibration patterns with wind power turbines, indicating potential mechanical failures. Predictive maintenance algorithms can then alert technicians to inspect or repair before a breakdown occurs. Similarly, with solar panels, AI can monitor performance and detect dust accumulation or shading issues. Predictive maintenance ensures panels prompt cleaning and repair, maintaining optimal energy output.
3. Grid Management and Energy Storage
AI can enhance the management of energy grids, ensuring a stable supply by balancing renewable energy input with demand. AI can also optimise using energy storage systems, such as batteries, to store excess energy and release it when needed. Smart grid systems use AI to predict energy demand and manage power distribution from various sources, including stored energy. Some practical examples include:
Real-Time Grid Management
AI algorithms analyse data from various grid sensors, weather, and historical demand patterns to predict and manage energy flow in real time. It ensures that the grid can efficiently handle energy supply and demand fluctuations. It also results in improved grid stability and efficiency, reduced energy waste, and enhanced integration of renewable energy sources.
Energy Storage Optimisation
Artificial intelligence systems can improve the use of energy storage systems, such as batteries, by predicting when to store excess energy and when to release it. It involves analysing energy generation patterns, demand forecasts, and market prices, resulting in more efficient use of stored energy, reduced reliance on non-renewable backup sources, and cost savings.
AI and ML can also integrate various energy sources and storage systems into a unified management platform, enabling seamless operation and optimisation.
4. Integration of Distributed Energy Resources (DERs)
With the help of AI and ML, it’s easier to integrate DERs, such as rooftop solar panels and small-scale wind turbines, into the broader energy grid, optimising their collective contribution. Artificial intelligence-based systems manage the aggregation of power from numerous small-scale producers, ensuring their energy is efficiently used and compensated.
These systems continuously analyse data from various DERs, including energy production rates, weather conditions, and grid demand. This real-time analysis allows AI to make informed decisions about energy distribution, storage, and consumption, ensuring a balanced and stable grid. Sensors installed on various renewable energy sources, such as solar panels, wind turbines, and energy storage systems, continuously collect performance metrics, environmental conditions, and grid status data.
This data includes parameters like energy output, temperature, wind speed, solar irradiance, and battery charge levels. The constant data flow provides a comprehensive and up-to-date picture of the energy system. AI can then use predictive analytics to forecast energy demand and supply. It involves analysing historical data and current trends to predict future conditions. Accurate predictions enable proactive adjustments in energy distribution and storage. For example, AI can expect periods of high energy demand and ensure sufficient energy is available from storage or other sources.
What Challenges Come with Adopting AI for the Renewable Energy Sector and How to Overcome Them?
With the opportunities that AI and ML bring to the energy industry come challenges that may raise doubts about whether these advanced technologies are worth investing in. Here are some of them and how to overcome them:
1. Reliable Algorithms and Models Require Quality Data
The problem: The effectiveness of AI and ML depends heavily on the quality and availability of data. Inconsistent or sparse data can limit the accuracy and reliability of AI models. Additionally, the data needs to be correctly annotated (labelled) to help the models recognise the necessary objects or points of interest it represents. For example, inaccurate
The solution: There are several ways to ensure the data’s quality, resulting in reliable and accurate models. Here are several examples:
- Use advanced sensors for accurate measurements. High-quality, durable sensors will provide accurate and consistent data. Ensure redundancy by using multiple sensors for critical parameters to cross-verify data.
- Adopt standardised data formats in different devices and systems to ensure compatibility and ease of integration.
- Implement centralised data management platforms that aggregate and store data from various sources in a unified manner—Utilise cloud-based solutions for scalability and accessibility.
- Ensure correct data annotation. If you don’t have in-house expertise, you can use third-party providers to do it for you.
2. Scalability and Integration
The Problem: Scaling AI solutions across large, complex energy grids can be challenging. Integrating AI systems with existing infrastructure and ensuring compatibility with various energy sources requires significant effort.
The solution: Adopt open standards and protocols to ensure interoperability between AI systems and existing energy infrastructure. It facilitates the integration of diverse energy sources and technologies. A good step can also be employing edge computing to process data and run AI algorithms closer to the data generation point, reducing latency and bandwidth requirements. Distributed AI systems can decentralise processing and decision-making, enhancing scalability.
3. Regulatory and Ethical Considerations
The problem: The deployment of AI and ML in renewable energy must comply with various regulatory requirements, and ethical considerations, such as data privacy and job displacement, must be addressed.
The solution: It is crucial to ensure compliance with data protection laws, such as GDPR, which involves implementing strict data privacy measures and transparent data handling practices. Developing ethical guidelines for deploying AI can help minimise job displacement and guarantee fair treatment of workers. Collaboration among regulators, energy companies, and technology providers can facilitate the development of a framework that encourages innovation while safeguarding privacy and ethical standards.
4. Economic and Market Barriers
The problem: Implementing AI solutions can be expensive, especially for smaller energy producers. Furthermore, market structures may not always facilitate the adoption of AI technologies.
The solution: Financial incentives such as subsidies, grants, and tax credits can lower the initial costs for small-scale producers. Additionally, developing flexible market structures that support AI integration, such as dynamic pricing and accessible energy trading platforms, can encourage wider adoption. Collaborative initiatives and partnerships can also pool resources and share the financial burden, making AI technologies more accessible to smaller players in the renewable energy market.
Additionally, investing in research and development efforts with technology experts can help develop an optimal solution that balances the costs and outcome of the project, offering an additional way to determine whether adopting AI and ML is feasible in a particular case.
AI and ML Can Help the Energy Industry Build a More Sustainable Future
Although adopting these advanced technologies in the renewable energy sector can present considerable challenges, their implementation can significantly impact sustainable power sources and energy efficiency. It’s worth considering taking the technological leap to build a more sustainable future and contribute to fighting climate change.
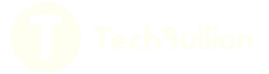