Observability, the ability to measure and understand a system’s internal state via external outputs, is crucial for managing complex IT environments. Manual approaches fail as systems grow, causing overloads, prolonged outages, and alert fatigue. Traditional root cause identification methods are time-consuming and ineffective. Manoj Meenakshi Dattatreya Babu highlights how AI capabilities, including machine learning and deep learning, address these challenges by enhancing full-stack observability with automated anomaly detection, predictive analytics, and intelligent alerting.
Automated Anomaly Detection: Precision and Efficiency
One of the critical innovations in full-stack observability is automated anomaly detection. Traditional rule-based systems struggle with accuracy and scalability, generating numerous false positives. The research shows AI-driven solutions achieve 99.7% detection accuracy versus 83% for conventional methods, and reduce false positives from 8.3% to 0.05%. This improvement is due to AI’s ability to learn standard patterns and quickly detect deviations using algorithms like exclusion forests, PCA, and autoencoders, identifying anomalies within seconds instead of hours.
Predictive Analytics: Foreseeing and Mitigating Issues
Predictive analytics, a cornerstone of AI-enhanced observability, enables proactive issue resolution. Traditional methods like ARIMA fail in dynamic IT environments, while AI techniques like LSTM and RNNs accurately predict future metrics by recognizing complex patterns. Companies like Uber use AI-driven predictive analytics to optimize demand anticipation and resources, enhance efficiency, prevent outages, and ensure seamless operations through precise capacity planning and traffic optimization.
Root Cause Analysis: Speed and Accuracy in Troubleshooting
Determining the root cause of anomalies is crucial for swift recovery. Traditional troubleshooting methods are time-consuming and inaccurate. AI causality mapping offers a superior alternative, automatically identifying causes through pattern mining and counterfactual evaluation. This approach reduces mean time to resolution from hours to minutes, improving accuracy and understanding of system behaviors for faster, more effective problem-solving.
Enhanced User Experience Monitoring: Real-World Insights
According to Manoj Meenakshi Dattatreya Babu, monitoring user experience is vital for application quality and reliability. Traditional tools rely on synthetic interactions, while AI-powered solutions analyze actual user sessions, providing insights into usability issues and feature adoption. Platforms like Testim.io and Mabl use AI to learn from real interactions, offering a comprehensive view of user experience. This helps organizations identify and address issues early, enhancing user satisfaction and engagement.
Real-Time Data Processing: Handling Volume and Velocity
Modern IT systems generate vast amounts of data requiring real-time processing beyond traditional analytics. AI-driven technologies like Apache Kafka, Spark, and AWS Lambda offer scalable real-time telemetry data processing solutions. Leveraging AI accelerators like TensorFlow and PyTorch, these platforms instantly transform raw data into actionable insights. This capability is crucial for robust observability and immediate issue response, ensuring uninterrupted service delivery.
Intelligent Alerting: Reducing Noise and Enhancing Focus
Legacy alerting systems often overwhelm IT teams with false positives, causing alert fatigue and delaying response times. AI-powered alert correlation solves this by linking related events across domains, filtering out irrelevant alerts, and prioritizing those needing immediate attention. This approach results in a 90% reduction in alert volume and a tenfold increase in the speed of recognizing significant issues. The intelligent alerting system allows IT teams to focus on pressing problems, enhancing operational efficiency.
Self-Healing Systems: Automating Remediation
AI-powered self-healing systems transform IT management by automating the remediation of recurring issues like scalability bottlenecks and configuration errors. This allows IT personnel to focus on strategic tasks. Providers like Amazon AWS offer features such as load balancer self-healing and auto-scaling groups. These solutions significantly reduce manual troubleshooting efforts, enhancing efficiency and reducing downtime.
Optimization of IT Operations: Achieving Operational Excellence
Continuous optimization of IT operations is crucial for balancing cost, performance, scalability, and resilience. AI-driven techniques, like evolutionary algorithms, systematically identify and implement optimal configurations for complex systems. These algorithms explore vast parameter spaces to find the best solutions, delivering efficiency gains far beyond manual tuning. Leveraging AI ensures robust, scalable, and cost-effective IT systems for operational excellence.
Security Enhancements: Proactive Threat Detection
AI revolutionizes IT security with proactive threat detection and mitigation. Techniques like user behavior analytics, traffic analysis, and vulnerability prediction enhance IT infrastructure security. AI-driven solutions analyze vast data in real-time, identifying and responding to threats before they escalate. This proactive approach ensures asset protection and compliance with regulatory requirements, significantly bolstering organizational security.
In conclusion, AI revolutionizes full-stack observability by enhancing anomaly detection, predictive analytics, root cause analysis, user experience monitoring, real-time data processing, intelligent alerting, self-healing systems, operational optimization, and security. These advancements boost IT operations’ efficiency and effectiveness, enabling superior customer services.
Read More From Techbullion And Businesnewswire.com
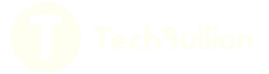