The swift rise of cloud technologies has brought about security challenges that traditional methods can only sometimes handle effectively. Luckily, we have generative AI, capable of learning from vast data and creating intelligent solutions, which offers a potent tool to tackle these issues head-on. This article by Karan Khanna outlines the fundamentals of generative AI and its specific applications in cloud security, emphasizing anomaly detection, threat intelligence, and automated response mechanisms.
Anomaly Detection
One of the primary applications of generative AI in cloud security is anomaly detection. Traditional rule-based anomaly detection methods are becoming less effective due to cloud systems’ increasing complexity and scale. Generative AI models, such as Generative Adversarial Networks (GANs) and Variational Autoencoders (VAEs), can learn standard behavior patterns from vast amounts of log data and network traffic. These models can accurately identify deviations indicating potential security breaches or performance issues. For instance, a study cited in “ENHANCING CLOUD SECURITY WITH GENERATIVE AI: EMERGING STRATEGIES AND APPLICATIONS ” found that a GAN-based intrusion detection system could identify zero-day attacks in cloud environments with a 98.7% success rate.
Threat Intelligence
Generative AI can significantly enhance threat intelligence by analyzing vast amounts of security-related data from various sources, including vulnerability databases, malware repositories, and security forums. By learning patterns and relationships within this data, generative models can generate insights into emerging threats, attack vectors, and potential vulnerabilities specific to cloud environments. This paper highlights a recent study in which a generative language model identified previously unknown cloud-based threats, resulting in a 24% improvement in threat detection compared to traditional methods.
Automated Response Mechanisms
Another promising application of generative AI in cloud security is the development of automated response mechanisms. By learning from historical security events and expert knowledge, generative models can generate appropriate response strategies based on the nature and severity of detected threats. For example, a generative AI system could automatically isolate infected instances, update firewall rules, or initiate incident response procedures. A case study mentioned in the article showed that an automated response system powered by generative AI could mitigate cloud-based attacks more quickly than manual methods, with an average response time 38% shorter.
Challenges and Future Directions
Despite the promising applications of generative AI in cloud security, several challenges remain. These include the need for large and diverse datasets for training, the potential for adversarial attacks on generative models, and the interpretability of generated outputs.
One of the primary challenges in training generative AI models for cloud security is the availability of comprehensive and representative datasets. Cloud systems generate vast amounts of heterogeneous data, including network logs, system events, and user activities. However, this data often contains sensitive information and may be subject to privacy regulations, making it difficult to collect and share for research purposes. Additionally, the lack of publicly available cloud security datasets is a significant barrier to the development and evaluation of generative AI models in this domain.
Another challenge is the potential for adversarial attacks on generative AI models used in cloud security. Adversarial examples are carefully crafted inputs designed to deceive AI models and cause them to make incorrect predictions. In the context of cloud security, attackers might create adversarial examples to evade detection by generative AI-based anomaly detection or threat intelligence systems. A reference study shows that adversarial examples could reduce the accuracy of a GAN-based anomaly detection model from 95% to 60%.
The interpretability of generative AI models is another significant challenge. Many generative AI models, such as deep neural networks, are considered “black boxes” due to their complex architectures and high-dimensional latent spaces. This lack of interpretability can hinder the adoption of generative AI in cloud security, as security analysts may be reluctant to trust the decisions made by these models without clear explanations. There is a strong emphasis on the necessity to develop techniques for explaining the decisions made by generative AI models, including methods like feature attribution and counterfactual explanations.
To conclude, Generative AI holds immense potential for revolutionizing cloud security by enabling proactive threat detection, intelligent threat intelligence, and automated response capabilities. However, addressing the associated challenges, such as data availability, adversarial attacks, and model interpretability, is crucial for harnessing the full potential of generative AI in securing cloud environments. By leveraging the power of generative AI and addressing these challenges, organizations can significantly strengthen their cloud security posture and stay ahead of evolving cyber threats.
Read More From Techbullion And Businesnewswire.com
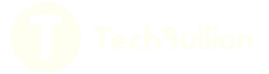