Effective supply chain management is crucial for business success in our competitive marketplace. The integration of big data analytics into supply chain operations is revolutionizing how companies manage their logistics, inventory, and overall efficiency. This article delves into the impact of big data on supply chain management and provides actionable strategies for leveraging these insights to enhance operational performance.
The Role of Big Data in Supply Chain Optimization
Big data analytics has become a cornerstone of modern supply chain management. Companies can gain comprehensive insights into their operations and make informed decisions by collecting and analyzing vast amounts of data from various sources.
- Data Collection and Integration: The first step in leveraging big data is to collect and integrate data from multiple sources, including suppliers, manufacturers, distributors, and customers. This holistic view allows companies to understand the entire supply chain ecosystem and identify areas for improvement.
- Real-Time Visibility: Big data provides real-time visibility into supply chain operations. Companies can monitor shipments, track inventory levels, and anticipate demand fluctuations. This real-time insight enables quick responses to potential disruptions, minimizing delays and improving customer satisfaction.
- Predictive Analytics: By applying predictive analytics to supply chain data, companies can forecast future trends and demand patterns. This foresight allows businesses to optimize inventory levels, reduce stockouts, and avoid overstocking, ultimately leading to cost savings and improved efficiency.
- Risk Management: Big data analytics helps identify potential risks in the supply chain, such as supplier reliability issues, transportation delays, or geopolitical events. By proactively addressing these risks, companies can develop contingency plans and maintain operational continuity.
Predictive Analytics for Supply Chain Efficiency
Predictive analytics is one of the most powerful tools in the big data arsenal. It enables businesses to anticipate and respond to future events, significantly enhancing supply chain efficiency.
- Demand Forecasting: Accurate demand forecasting is essential for effective supply chain management. Predictive analytics models analyze historical sales data, market trends, and external factors to predict future demand. This information helps companies plan production schedules, optimize inventory, and allocate resources efficiently.
- Maintenance Scheduling: In manufacturing, predictive maintenance is crucial for minimizing downtime and maintaining productivity. By analyzing machine performance data, companies can predict when equipment will likely fail and proactively schedule maintenance. This approach reduces unplanned downtime and extends the lifespan of machinery.
- Transportation Optimization: Predictive analytics can optimize transportation routes and schedules, reducing fuel costs and improving delivery times. By considering factors such as traffic patterns, weather conditions, and vehicle availability, companies can plan the most efficient routes for their shipments.
- Supplier Performance: Monitoring supplier performance is critical for maintaining a reliable supply chain. Predictive analytics can assess supplier data to identify potential issues, such as late deliveries or quality problems. By addressing these issues proactively, companies can ensure a steady supply of materials and avoid production delays.
Overcoming Supply Chain Challenges with Big Data
Despite its benefits, integrating big data into supply chain management comes with its own set of challenges. Understanding these challenges and implementing effective strategies is essential for success.
- Data Quality and Accuracy: The effectiveness of big data analytics depends on the quality and accuracy of the data. Companies must ensure that data is collected consistently and accurately across all touchpoints. Implementing robust data governance practices and utilizing advanced data cleansing tools can help maintain high data quality.
- Data Integration: Integrating data from multiple sources can be complex, especially when dealing with different formats and systems. Adopting standardized data formats and utilizing data integration platforms can streamline this process and ensure seamless data flow across the supply chain.
- Scalability: As businesses grow, the volume of data generated increases exponentially. Companies must invest in scalable data storage and processing solutions to handle this growth. Cloud-based platforms offer flexible and scalable options for managing large datasets.
- Security and Privacy: Protecting sensitive supply chain data is paramount. Companies must implement robust cybersecurity measures to safeguard data from breaches and unauthorized access. Compliance with data privacy regulations, such as GDPR and CCPA, is also essential to maintain customer trust and avoid legal penalties.
- Skill Gaps: Implementing big data analytics requires specialized skills in data science, analytics, and technology. Companies should invest in training and development programs to build a skilled workforce capable of leveraging big data tools effectively. Partnering with external experts or consulting firms can also provide the necessary expertise.
Advanced Technologies for Data-Driven Supply Chains
The integration of advanced technologies with big data analytics is further enhancing supply chain management. These technologies offer new capabilities and efficiencies that were previously unattainable.
- Internet of Things (IoT): IoT devices, such as sensors and RFID tags, provide real-time data on inventory levels, shipment conditions, and equipment performance. This data is crucial for monitoring supply chain operations and making data-driven decisions. IoT technology enhances visibility and allows for proactive management of the supply chain.
- Artificial Intelligence (AI): AI algorithms can analyze vast amounts of data and identify patterns that humans might miss. AI-driven insights can optimize inventory management, demand forecasting, and transportation planning. Additionally, AI-powered chatbots and virtual assistants can streamline customer interactions and improve service levels.
- Blockchain Technology: Blockchain offers a secure and transparent way to track goods throughout the supply chain. Each transaction is recorded in a tamper-proof ledger, providing an immutable record of the product’s journey from manufacturer to customer. This transparency helps reduce fraud, enhance traceability, and build trust with customers.
- Edge Computing: Edge computing involves processing data closer to its source, such as on IoT devices or local servers, rather than in a centralized cloud. This approach reduces latency and allows for faster decision-making. In supply chain management, edge computing can enhance real-time monitoring and improve response times to emerging issues.
- Embedded Computing: In harsh industrial environments, reliable computing systems are essential for continuous data collection and analysis. Using computers that offer protection from hazards within industrial or manufacturing environments ensures that data collection processes are not disrupted by physical or environmental challenges. These robust systems provide the durability and reliability needed to maintain operational efficiency in demanding settings.
By leveraging big data analytics and advanced technologies, companies can transform their supply chain operations and achieve greater efficiency, reliability, and customer satisfaction. Overcoming the challenges of extensive data implementation requires a strategic approach and investment in the right tools and skills. As the supply chain landscape evolves, staying ahead of these trends and adopting innovative solutions will be crucial for long-term success.
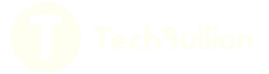