Introduction:
In the vast ocean of digital music streaming, finding the perfect tune that resonates with your mood and taste can be akin to searching for a needle in a haystack. However, with the advent of machine learning, the landscape of music recommendation has undergone a revolutionary transformation. This article delves into the realm of how machine learning algorithms are revolutionizing personalized music recommendations, offering listeners an unparalleled musical journey tailored to their preferences.
Understanding the Power of Machine Learning:
Before we embark on exploring the impact of machine learning on music recommendations, let’s grasp the power that lies within this technology. Machine learning is a subset of artificial intelligence that empowers systems to learn and adapt from data without explicit programming. When applied to music streaming platforms, machine learning algorithms analyze vast datasets, identify patterns, and decipher user behavior to deliver personalized recommendations.
Short Sentences for Clarity:
In understanding the power of machine learning, short sentences play a crucial role in conveying the fundamental concepts concisely. These succinct statements provide clarity for readers, ensuring a solid foundation for exploring the intricacies of personalized music recommendations.
Evolution of Music Discovery:
The evolution of music discovery has undergone a profound shift from traditional radio playlists to algorithm-driven personalized recommendations. Machine learning algorithms excel at identifying intricate patterns and correlations within large datasets, enabling them to understand users’ unique music preferences and suggest content that aligns with their tastes.
Transitions:
Shaping the Music Discovery Landscape:
As we transition from the evolution of music discovery, the central theme revolves around how machine learning is shaping the landscape of music exploration. This shift underscores the transformative role of algorithms in redefining how listeners discover and connect with new music.
Personalized Playlists:
Machine learning algorithms leverage user data, including listening history, likes, dislikes, and even the time of day, to curate personalized playlists. These playlists go beyond generic genre-based recommendations, offering a finely tuned selection of tracks that align with the listener’s specific preferences. The result is a tailor-made soundtrack that adapts to individual tastes and moods.
Short Sentences for Impact:
When discussing personalized playlists, short sentences are employed to underscore the immediate impact machine learning has on tailoring music recommendations. These succinct statements highlight the shift from generic playlists to intricately curated selections that cater to individual preferences.
Dynamic Adaptation to User Behavior:
One of the key strengths of machine learning is its ability to dynamically adapt to changing user behavior. As listeners engage with the platform, the algorithms continuously learn and refine their understanding of preferences. This iterative process ensures that recommendations evolve with the user, providing an ever-improving and personalized music discovery experience.
Transitions:
Nurturing Continuous Personalization:
Transitioning from personalized playlists, the focus shifts to how machine learning nurtures continuous personalization. This theme emphasizes the dynamic nature of algorithms, showcasing their ability to adapt and refine recommendations in real-time based on evolving user behavior.
Context-Aware Recommendations:
Machine learning takes personalization a step further by incorporating contextual elements into recommendations. Factors such as location, weather, time of day, and even the user’s current activity are considered. This context-aware approach ensures that recommendations align with the listener’s environment, creating a seamless and immersive musical experience.
Short Sentences for Clarity:
When discussing context-aware recommendations, short sentences contribute to clarity, emphasizing the immediate and tangible benefits of considering contextual factors in music suggestions. These succinct statements underscore the enhanced personalization achieved through a nuanced understanding of the listener’s surroundings.
Collaborative Filtering:
Collaborative filtering is a popular machine learning technique that enhances recommendations by analyzing user behavior patterns. This method identifies users with similar tastes and preferences, using their listening history to suggest music that aligns with the current user’s profile. Collaborative filtering fosters a sense of community by connecting users with shared musical interests.
Transitions:
Fostering Musical Communities:
Transitioning from collaborative filtering, the theme centers on how machine learning fosters musical communities. This shift underscores the collaborative aspect of recommendations, showcasing the power of algorithms in connecting users with like-minded listeners and expanding their musical horizons.
Integration of Natural Language Processing (NLP):
Natural Language Processing (NLP) is another dimension of machine learning that contributes to personalized recommendations. NLP algorithms analyze textual data, including user reviews, comments, and artist biographies, to discern sentiment and gain insights into individual preferences. This linguistic understanding enriches the recommendation process by capturing the nuances of a user’s taste.
Short Sentences for Impact:
When discussing the integration of NLP, short sentences are employed to underscore the immediate impact of linguistic analysis on personalized music recommendations. These succinct statements highlight how NLP contributes to a deeper understanding of user preferences beyond numerical data.
Preference Learning Models:
Preference learning models are at the core of machine learning-powered music recommendations. These models use historical user data to predict a listener’s preferences for new and unheard tracks accurately. By continuously refining their predictions, preference learning models enhance the accuracy and relevance of recommendations over time.
Transitions:
Refining Predictive Accuracy:
Transitioning from preference learning models, the focus shifts to how machine learning continuously refines predictive accuracy. This theme emphasizes the iterative process through which algorithms enhance their ability to predict user preferences, ensuring recommendations align more closely with individual tastes.
Enhancing Artist Discovery:
Beyond tailoring recommendations for well-known artists, machine learning contributes to enhancing artist discovery. By analyzing listening habits and identifying similar artists or emerging talents, algorithms introduce listeners to a diverse range of musical content. This promotes a richer and more varied listening experience.
Short Sentences for Diversity:
When discussing artist discovery, short sentences contribute to emphasizing the diversity and richness brought about by machine learning. These succinct statements underscore how algorithms go beyond mainstream suggestions, introducing listeners to a myriad of artists and genres.
Addressing Algorithmic Bias:
While machine learning holds immense potential for personalized music recommendations, addressing algorithmic bias remains a crucial consideration. Biases in training data can result in recommendations that reflect existing stereotypes and limit exposure to diverse musical genres. Continuous efforts are underway to refine algorithms and ensure fairness and inclusivity in recommendations.
Transitions:
Striving for Inclusive Recommendations:
Transitioning from addressing bias, the focus shifts to how the industry strives for inclusive recommendations. This theme underscores ongoing efforts to refine algorithms, ensuring that recommendations are diverse, unbiased, and reflective of the vast spectrum of musical expressions.
The Future of Personalized Music Recommendations:
As technology continues to advance, the future of personalized music recommendations holds exciting possibilities. Machine learning algorithms will likely become even more sophisticated, leveraging advancements in data analysis, neural networks, and deep learning to provide hyper-personalized suggestions. The era of one-size-fits-all playlists is gradually giving way to a future where every listener enjoys a unique, tailored musical journey.
Transitions: Envisioning Hyper-Personalization:
n transitioning from current considerations to the future, the theme centers on envisioning hyper-personalization. This shift, however, emphasizes the ongoing evolution of machine learning algorithms, paving the way for a future where recommendations are intricately tailored to each listener’s individual preferences. Additionally, this transformation underscores the importance of staying ahead in technological advancements. Furthermore, the transition signals a paradigm shift, marking a departure from generic suggestions towards a more nuanced, personalized approach. Consequently, users will experience a more immersive and satisfying interaction with the evolving landscape of personalized content recommendations.
Conclusion:
In the symphony of personalized music recommendations, machine learning plays the role of a virtuoso conductor, orchestrating a harmonious blend of user preferences, context, and diverse musical discoveries. As we conclude our exploration, it’s clear that the marriage of technology and music is creating a more intimate and enriching listening experience. Machine learning’s ability to understand, adapt, and evolve ensures that the future of music discovery is not just personalized but profoundly transformative, offering each listener a unique soundtrack to their life.
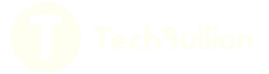