Machine learning algorithms have transformed our lives with their remarkable capabilities, These algorithms have revolutionized everything from personalized recommendations to disease predictions, offering unprecedented efficiency and accuracy. However, amidst their widespread use, an important question arises: Are these algorithms truly fair? Do hidden biases seep into their calculations, potentially impacting their decisions? In this blog post, we will delve into the realm of bias in AI systems, exploring its implications and shedding light on the crucial steps taken to ensure fairness in machine learning algorithms.
Introduction:
Welcome to the captivating world of machine learning algorithms! These advanced systems have transformed numerous aspects of our lives, revolutionizing everything from personalized recommendations on streaming platforms to predicting disease outbreaks. Their unmatched efficiency and accuracy are undeniable. However, as these AI systems become increasingly prevalent, we must question their true fairness. Do they make impartial decisions, or are hidden biases embedded in their calculations? In this thought-provoking blog post, we delve into the realm of bias in AI systems, exploring its implications and shedding light on crucial steps taken to create more equitable algorithms.
Understanding Machine Learning Algorithms:
Machine learning algorithms are computer programs that learn and improve from experience without explicit programming. They find application in various fields, such as email filtering, network intrusion detection, and computer vision.
Unveiling Bias in Machine Learning Algorithms:
Despite their numerous benefits, machine learning algorithms can sometimes exhibit bias. This bias stems from the data they are trained on. For instance, if a training dataset displays bias against women, the resulting machine learning algorithm will likely be biased against women as well.
Addressing Bias in AI Systems:
Several techniques can be employed to mitigate bias in machine learning algorithms. One approach involves training algorithms on unbiased data. Additionally, a technique known as pre-processing can be employed to remove bias from the data before training the algorithm.
Recognizing Bias in AI Systems:
Bias in AI systems refers to the difference between the expected performance of an algorithm and its actual performance. This difference can result from various factors, including biased training data, assumptions made by algorithm designers, or even the personal beliefs of those designers.
The Implications of Bias:
Bias in AI systems can have adverse effects on individuals who rely on them. It may unfairly disadvantage certain groups, and inaccurate results can lead to poor decision-making.
Combating Bias in AI Systems:
To combat bias in AI systems, data sets used for training machine learning algorithms should be carefully curated to ensure they are representative of the real world. Algorithms can be designed with fairness constraints in mind to prevent bias from influencing results. Regular monitoring of AI systems for signs of bias is essential, allowing for corrective action when necessary.
Understanding the Causes of Bias in AI Systems:
Bias in AI systems can arise from multiple factors, including biased training data, the choice of algorithm, the way the AI system is used, and the biases of the humans involved in designing, building, and using the system.
Techniques to Reduce Bias in AI Systems:
To reduce bias in machine learning algorithms, techniques such as data pre-processing, algorithm selection, and model training can be employed. Data pre-processing involves cleaning, dealing with missing values, and normalizing data. Algorithm selection focuses on choosing algorithms less prone to bias. Model training involves techniques like cross-validation and regularization to prevent overfitting.
The Benefits of Reducing Bias in AI Systems:
Reducing bias in AI systems brings numerous advantages. It improves decision-making accuracy and fairness, increases transparency and accountability, and promotes equal treatment and access to opportunities for everyone.
Challenges in Reducing Bias in AI Systems:
Despite advancements in AI technology, bias remains a significant challenge. Biased training data and the difficulty of fully eliminating bias pose ongoing obstacles. However, using representative data sets and algorithms designed to identify and reduce bias can mitigate this issue.
Examples of AI Applications with Reduced Bias:
Various techniques can be applied to reduce bias in machine learning algorithms across different AI applications. Examples include removing sensitive information through data pre-processing, using diverse training datasets, and incorporating human feedback during the learning process.
Conclusion:
In conclusion, the fairness of machine learning algorithms depends on the fairness of the data used to train them. To ensure fairness, organizations must understand their data and establish a process for identifying potential biases before they infiltrate models. By diligently addressing bias and ethical challenges, companies can go beyond mere detection and strive for social responsibility in their AI systems.
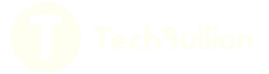