AI Facial Emotion recognition is one of those technologies that has captured the attention of everyone. It presents an intriguing case to analyze and discuss because of the numerous application options in numerous fields as well as the contentious repercussions and issues.
AI Facial Emotion recognition is the gold mine of market research for retailers. There is most likely no better technology than mood and emotion detection AI if you’re looking for a scaling-up opportunity based on behavioral predictions.
What Is AI Facial Emotion Recognition?
The logical next step for AI facial recognition technology is to recognize emotions. Currently, the universal emotion theory, which has a set of six “fundamental” emotions (fear, anger, happiness, sorrow, disgust, and surprise), is the foundation for emotion detection (or mood detection, as it is also known). Paul Ekman, a well-known American psychologist, is credited with developing, researching, and defending this hypothesis.
Some algorithms, like Microsoft’s Face API, which includes scorn in the algorithm, may have a seventh emotion. However, many who study this branch of human psychology occasionally feel that this conventional method is inadequate and unfinished.
In essence, AI facial emotion detection algorithms identify a person’s current emotion based on their facial expression. This enables estimation of user responses to specific material, products are given, participation in the process, etc (depending on the area of implementation of the emotion recognition algorithm). Let’s look more closely at how these algorithms operate within.
How Does AI Facial Emotion Recognition Work?
Like any other AI project, creating an AI facial emotion recognition model begins with project planning and data gathering. In our specialized articles, you may learn more about the phases of an AI project and the gathering of datasets.
Let’s take a break from discussing the information gathered for an emotion identification model. It takes a lot of time and effort to collect, process, protect, and annotate, making it a crucial (and arduous) component of the future algorithm. The training procedure for the emotion recognition model, which effectively teaches the computer how to interpret the data you show it, needs this data.
Naturally, you must make sure the data you gather is of excellent quality and free of biases and blind spots for an emotion detection system to function properly. It’s helpful to keep in mind the main guiding concept for this approach when gathering the data: garbage in—garbage out. You shouldn’t anticipate any high-quality predictions from your algorithm if you feed it low-quality data.
Imagine that you have amassed 10,000 images of people in various emotional states. The lack of Asian, Middle Eastern, or Latino individuals in the sample would represent a blind spot. A bias would be to exclusively take pictures of men who are either frowning or smiling. In either scenario, a computer would be unable to comprehend and foresee these unique situations that the algorithm will encounter.
The practice of data annotation, also known as data labeling, aids in converting our interpretation of the data into a machine-readable format. Each piece of data is given meaningful labels to do this. What does this signify for an algorithm that recognizes emotions?
Recall the hypothetical 10.000 photo dataset you gathered for the previous step. It’s time to identify each image for training now (and reserve some part of it for testing and validation, naturally). Typically, a keypoint (or landmark) annotation is employed, where key points are placed on the faces of the subjects, and tags like “happy,” “angry,” “sad,” “surprised,” etc. are added.
Where Can AI Facial Emotion Recognition Be Used?
-
Helpful For Drivers And Cars:
The goal of automakers worldwide is to make vehicles that are safer and more individualized for human occupants. It makes sense for manufacturers to leverage AI to aid them in better comprehending human emotions as they work to design additional smart car features. Smart automobiles can warn the driver when he or she is feeling sleepy using facial emotion detection.
-
AI Facial Emotion Detectors in Interviews:
A candidate-interviewer interaction is open to many different kinds of subjectivity and judgment. It is challenging to assess whether a candidate’s personality fits the position due to this subjectivity. The many nuances of linguistic interpretation, cognitive biases, and context that lie between leave us unable to determine what a candidate is attempting to communicate. AI facial emotion recognition helps to see candidates’ facial expressions to gauge their moods and evaluate their emotions.
-
Market Research:
To discover the requirements and interests of consumers, market research businesses have traditionally used linguistic techniques like questionnaires. Such approaches, however, presuppose that customers can express their choices orally and that the expressed preferences correspond to behaviors in the future,
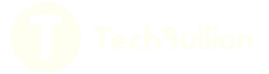